People Analytics is one of the hottest topics there is in HR at the moment. A data revolution is starting to make companies radically rethink their approaches to attracting, retaining and developing talent.
Technology is transforming the kind of qualitative research that underpins employer branding and employee engagement strategies, providing the ability to generate instant insights that would have previously taken months of work to produce.
My guest this week is Andrew Marritt from Organization View. Andrew is a pioneer in the field of text analytics for HR and has some fascinating insights into the potential for data in the talent space.
• In the interview we discuss:
• The relevance of Big Data for HR
• The power of using technology to bring meaning to the free text data found in performance reviews and employee surveys on a massive scale
• How free text analytics can help to develop subtle differences in employer brand messaging to give maximum appeal across multiple target audiences
• The potential for real time analysis of job applications to generate automated smart follow up questions, particularly in the graduate recruitment space
Andrew also share his thoughts on the application of text analytics to email and workplace collaborations systems to spot conversational trends within organizations
Subscribe to this podcast in iTunes
Recruiting Future with Matt Alder - What's Next For Talent Acquisition, HR & Hiring?
Ep 53: Unique Insights From People Analytics
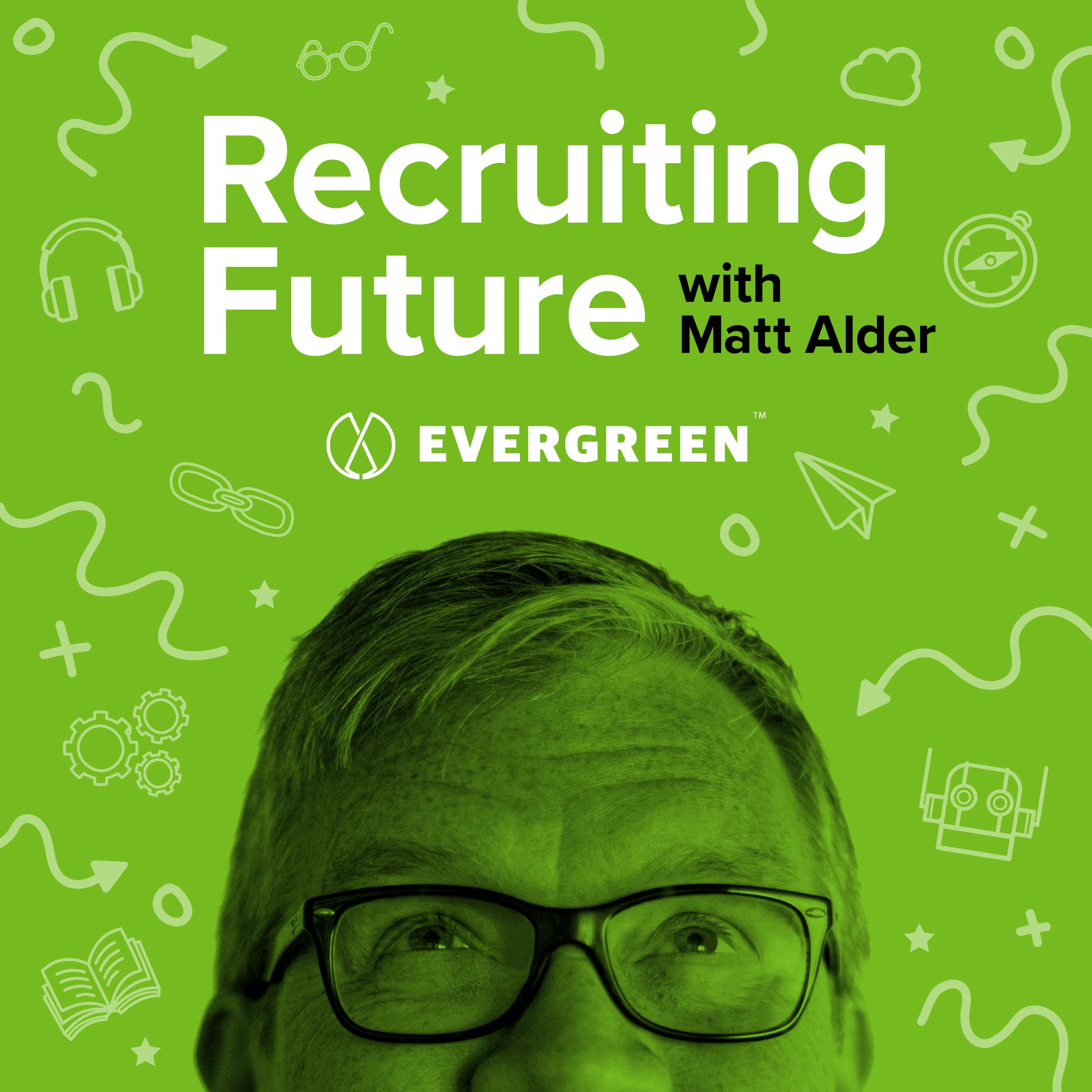
People Analytics is one of the hottest topics there is in HR at the moment. A data revolution is starting to make companies radically rethink their approaches to attracting, retaining and developing talent. Technology is transforming the kind of qualitative research that underpins employer branding and employee engagement strategies, providing the ability to generate instant insights that would have previously taken months of work to produce. My guest this week is Andrew Marritt from Organization View. Andrew is a pioneer in the field of text analytics for HR and has some fascinating insights into the potential for data in the talent space. • In the interview we discuss: • The relevance of Big Data for HR • The power of using technology to bring meaning to the free text data found in performance reviews and employee surveys on a massive scale • How free text analytics can help to develop subtle differences in employer brand messaging to give maximum appeal across multiple target audiences • The potential for real time analysis of job applications to generate automated smart follow up questions, particularly in the graduate recruitment space Andrew also share his thoughts on the application of text analytics to email and workplace collaborations systems to spot conversational trends within organizations Subscribe to this podcast in iTunes
- Duration:
- 28m
- Broadcast on:
- 13 May 2016
- Audio Format:
- other
Support for this podcast comes from CXC Corporate Services, part of CXC Global. They are the leading global advisor on contingent workforce solutions, helping companies reduce cost, mitigate risk and improve multi-country compliance across their contingent workforces and supply chains. To find out more about how CXC Corporate Services can help you, please visit www.CXCcorporateServices.com. That's www.CXCcorporateServices.com. CXC, facilitating the future of work. There's been more of scientific discovery, more of technical advancement and material progress in your lifetime and mind at all the ages of history. Hi everyone, this is Matt Alder. Welcome to episode 53 of the Recruiting Future podcast. People Analytics is the hot topic in HR at the moment. Technology is fueling a data revolution that is starting to make companies radically rethink their approaches to attracting, retaining and developing talent. Technology is revolutionizing the kind of qualitative research that underpins employer brand and employee engagement strategies with the ability to generate instant insights that would have previously taken months of work to get. My guest this week is Andrew Marat of People Analytics Specialist Organization View. Andrew is a pioneer in the field of text analytics for HR and has some fascinating insights into the potential for data in the talent space. Hi Andrew and welcome to the podcast. Good morning Matt, how are you? I'm very good. I understand it's a very snowy Monday morning where you are. Yes, it's the weather's taking a turn like a week ago. It was t-shirt and shorts weather and now it's snow. The joy is for being in Switzerland and spring. Absolutely. Could you tell us a bit about yourself, your background and what you do? Certainly. I'm Andrew Marat. I'm the founder of a prominent European People Analytics firm called Organization View. I founded Organization View six and a half years ago after first a career in the management consulting sector and then mostly Nazis working in big global HR departments, running technology and data-driven projects. The emphasis for starting Organization View is really a frustration of seeing how data and analysis was being managed in HR. The time I was sitting on the HR management team of a large international and every month we would have people coming and presenting data to us and every month I would get more and more frustrated about the responsiveness and also to some degree the quality of that analysis. In 2010, I decided to form a business with really nothing granted and sought out data for HR. That's where I'm at the moment. We do three different things. We are a business which has a consultancy approach working with large international clients helping provide additional capacity and capability to their analytics functions. Our clients tend to be already quite advanced in analytics and we're able to bring them probably more advanced skills than they have in house. Secondly, I do quite a lot of work teaching analytics and finally, we have a product called Work commentary which is around the employee feedback market and that uses a lot of the advanced algorithms that we've developed with clients but packages top into a product which is available for anyone regardless of how mature they are within their analytics capability. That's us. We're doing very well as I suspect everybody is in the analytics space at the moment. It's particularly the advanced text analytics that I was interested in talking about. We've met many times normally at a conference somewhere in the world where we don't live. You kind of showed me the text analytics and I thought it was amazing and I thought the applications of it in the HR space were quite considerable. Could you just talk everyone through what it is you've developed and what it does and why it's different? Let me take a step back and let's preface it around the conversation about big data which is probably a phrase which is less used these days than it was one two years ago. There were always multiple characteristics to big data and the name really drew attention to the size aspect. The size is relatively important but of the three or four challenges that Ghana initially identified I think it's certainly from an HR perspective it's the least important. One of the big problems that the big data was trying to, those sort of phrase around big data was trying to cover was the fact that the data has a lot of different types. I think there's a phrase about veracity. If we look at data and look at the growth of data, actually a lot of that data is non numerical data. It's data in vast amounts of text, it's vast amounts of images, it's vast amounts of video and it's the difficulty of dealing with this data which is not in a traditional database structure which has always been the hard part for HR and I think overlooked. If we think about HR there's a large amount of text data that sits within HR systems and also that we want to have a look at in the broader scheme so open comments within survey questions. We're even doing some work at the moment on email content. The approach of text analytics is really to use a variety of techniques to try and provide structure into that text. There are two different things that you might want to do with a piece of text. Firstly you might want to categorize it to some degree explaining what the person is talking about or the writer is talking about and secondly you might want to score it against some score regardless of whether it's an emotion or whether it's something more simple like sentiment. The categorization is what we focus on most. You can think about the algorithms trying to automatically tag some content to identify, to help the navigation and help that approach of understanding that. Once you've got structured data in that sense the tags, the entities, the topics you can then do a whole variety of statistical analysis with it and be able to deal with that text and scale. I think it's a really interesting approach and I think what really brings it alive is the applications that it can be used for. Could you talk us through some of the ways that you're using this or you see this being used in HR, in employer brand, in recruitment, etc. Sure. There's three things that we've done over the last couple of months and before that I think are good examples. The first of which is to look at text within existing HR systems and one of the areas which I've been conscious had held a lot of value for a long time was performance management comments. If we look at 360 appraisals, we've traditionally done analysis of potentially what rating people have been given depending on the organization. You might have to do that down to competency level and that's generally a one to five score. However, that score either at an overall level or even down to competencies is backed with a lot of text, with a lot of open text. We've done some analysis recently looking at 360 comments and looking at how people describe themselves and how their managers describe them. We've also done that in terms of gender. Looking at gender specific language use, do men exhibit some form of bias when they're talking about or managers in form of bias when they're talking about their employees in terms of gender bias, do women and men talk about themselves differently when they're giving their self ratings. We found some really interesting analysis of that. We took a data set which I think originally had around 30,000 rows of data, so 30,000 individual comments. You break this out into first of all looking at the topics and the types of the way those topics are being used and then doing analysis there. It quickly does become quite a large data problem. We were having a flat file from those 30,000 comments which was close to a gigabyte in size, so way past the ability to analyze it in Excel. We're able to pull out some really important information for the client in terms of, as I say, but how men and women describe themselves during the performance process and also how their managers describe them. One of the things that we talk about or that we've spoken about a lot on this podcast with other guests is employer branding and how people can get stories from their employees or attitudes from their employees or understand how their employees feel about the brand of the company or what it's like to work at the company, how they can do that at scale. Is this something that your tool could address? One of the things that I used to do when I was looking after employee brands and one of my roles in Nauties was in charge of employee level employee marketing at UBS, the Swiss Bank. We wanted to try and understand that those employee messages so that they were appealing to candidates, but also they had a strong match with reality. That reality differs depending on who you are in the organization. What it means to work for an organization if you're sitting in technology is very different than if you're in the salesforce or in the banking terms in front office. We had conducted quite a lot of research at UBS using traditional qualitative approaches to try and understand what that messaging was about. This has perceived employee brand messages from the employees. You can also do it to some degree as well with external markets, but the approach is remarkably similar. Historically, it's been about doing qualitative sessions, interviews, focus groups, that type of activity. One of the things that is common across any of that qualitative interview is that it's expensive. It's very time consuming, not only to conduct the interviews, but then to do the coding. What we've done with our approach and the work commentary tool uses this quite a lot within its applications into employing propositions is to ask employees at scale and to a lesser degree, ask external people whether they're through the recruitment process or even further outside their views on working on the organization. Our approach for internal employees is really three questions. First of all, a net promoter score question saying would you recommend your company as a place to work for friends or acquaintances? You then ask two open text questions. One, if a friend or acquaintance did come to you looking for your views on working for this organization, what would you say the positive things would be? A second question about what would you caution them about? Within that text, we get generally several sentences worth of content. We're picking up topics automatically, multiple topics from there, and looking to do this potentially even across the whole organization. Looking at identifying, let's say, three or four different topics, per statement, and from five, ten thousand different people. From there and combining that information, those tags with information about who the employee is, their gender, where they're working in the organization, how long they've been working in the organization. A host of other bits of information. We can then start building quite strong segmentation models to say these are the key messages that are resonating with employees of a certain type, a certain group. You can identify, use this approach, what are the messages that you would use to position working in the technology function, and what the messages that be useful in the front office business. We can do the coding with the algorithms that built into work commentary almost in real time. Certainly on those type of questions, we've got quite a lot of experience and tens, potentially hundreds of thousands of rows of data at the moment. We're finding that for the vast majority of it, we can code this in real time. Our approach is to do further tuning at the question level for each employer, because there may just be something that appeals to working in one organization that we haven't seen within the data before. The approach we're using learns the topics from the data itself, rather than having a predefined model. Obviously, we use previous experience to continue re-refined, but it's quite clear that reasons for wanting to work or things that put off employees about some organizations differ depending on the organizations and having an organization-specific model works. We're able to do what would have taken several months in a qualitative study and narrow it down to several days, and to do it at a much greater scale than you could ever do in a qualitative study. From what you're seeing so far, what's the most surprising insight that you've got from analyzing this kind of data in this kind of way? I think one of the things is a difference between groups of employees, which are potentially far away from HR teams and from managers within organizations, and their views compared to what the managers think. Let's take an example of retail. Retail is an industry where certainly now in the UK with the recent races of minimum wage, it's pretty close to everybody in retail. The shop floor will be paid pretty close to minimum wage, and managers will feel that salary and pay is a negative factor for people in that organization in those type of organizations. What we find though is that pay and those sort of functional parts of the salary package or even employment offer tend to be much more those working in head office and potentially in higher paid jobs are more likely to find issues around that, and that people on the shop floor, albeit they're the lowest paid within the organization, they often think that the pay is one of the good things about working for the organization. I think this is possibly because the alternatives all pay around the same, and therefore you can't differentiate on pay so much. It's interesting though you can potentially differentiate on non-pay items such as the ability to change your own hours, the quality of the staff discount scheme, those type of things make a lot of difference, but it's often getting quality of evidence to to change some of these biases that especially managers in head office think about the organization and the people who work for them. Yeah, I think that's one of the most interesting things. Yeah, that's really interesting actually. I think it just just shows the kind of thing that this type of data can shed light on. Last question, and this is really a question about where you think this is going to go in the future. It's interesting that in the last couple of weeks, Facebook have come out and shared their 10-year vision about where they're going, and a big part of what they were doing is artificial intelligence, and the first iteration of that is text-based spots in Messenger, which they launched last week, and the feedback so far isn't great. But I think that's an interesting development area for smart text interfaces. What's your vision of the future for this type of technology? I think it's inevitable that text becomes a large part of analysis of the sort of advanced levels of analysis. I think that HR will start to bring in tools that are able to process large volumes of text quite quickly. There's some really interesting examples outside the employee value proposition and employee feedback space that we've been doing. So we've been doing a piece of work with one big organization where the CEO asked his employees to communicate with him directly about their fears, concerns, and feedback for the executive team. And of course, got a large volume of emails needing to be able to deal with that and understand them at scale. So yes, they're reading and dealing with each individually, but to be able to provide some form of identification of patterns, for example, we've been doing some work looking at those emails and trying to effectively pattern spot, which parts of the organization are people more likely to be talking about certain topics? What are the breakdown of topics? How does it link to other information? I think we'll also start listening to parts of data that we probably haven't traditionally thought of within HR systems. So internal discussion groups or Yammer or whether it's on share points, those type of things. I think we're already in conversation with several clients who want to do effectively live dashboardsing on topics within there and to use that information and sort of holistic one to be able to understand patterns. I have to say in no instance are we doing things that would say Jim is increasingly talking about X, we're looking at aggregate levels and saying has there been I think it's more like a Twitter, what are the trending topics here and have we seen splits? And I think that type of system could be used quite quickly to provide early warnings to senior management of what's going on in the organization. If you find that your Latin American business starts talking about a certain type of client and that's new, then you might want to look at increasing the attention into that area. We've been doing some work on shared services to understand the types of calls and communications that are coming through so that we can then predict who's most likely to be calling the helpline or to be emailing the helpline. So for example, if you see that three months in to an organization, people start asking about filling expense forms in, then maybe you want to push out some training at two months to talk about expense forms and that would you look at the ROI there by the reduction on calls to the help desk. If you think of a help desk as sort of a last case where people can't find the information themselves, then spotting where you want to be proactive is potentially reducing the need for those people to contact you. So I see some of that aspect coming through quite quickly. We've also got one client where we're putting in place some in feedback. There's rules depending away you are that if an employee mentions a certain topic within any type of feedback, you need to be able to respond to it and we can put triggers that say spot for people mentioning bullying in terms of in an employee survey and let's define the process there to act on this quickly. So you can think about using text as well as diverting attention to where it's most needed and doing it quickly. So I think we'll see that coming through importantly one of the things we've done within well commentary is realize that because we can deal with texts so well, we don't need to ask as many traditional survey questions. So I mentioned before a three question survey which would really capture employee value proposition in a detailed manner. This has been able to deal with text in this way means that you can rethink the user interface and therefore the user experience. We could potentially think of application forms especially I've spent a lot of time working in the graduate market where historically students have pages of application forms to complete and thinking how can we use text and understanding text to radically reshape that user interface and the user experience to increase the user experience and potentially like the sort of testing where depending on how you answer one question you get asked further questions. I think we potentially could start to think about application forms where the application system is constantly reviewing the information you've got whether it's open text and asking follow-up questions. So I could see an instance where you link to a CV or enter a CV and the technology reviews that CV and asks you relevant follow-up questions based on clarification and that could therefore go several steps of the selection process. There's some really great firms doing work in there, you know, a text kernel is doing some really really great work on semantic understanding of text from the job piece and it's not an area. We specialize in but I think we'll start to see this where the ability to understand text in real time dramatically shifts the way that we ask questions and we don't need to ask questions in a way that it was traditionally database-friendly. Andrew, thank you very much for talking to me. It's a pleasure Matt because speaking to you as always. My thanks to Andrew Barat. You can subscribe to this podcast on iTunes or your podcasting app of choice, just search for a recruiting feature. You can find all the past episodes of the show at www.rfpodcast.com. On that site you can subscribe to the mailing list and find out more about working with me. Thanks very much for listening. I'll be back next week and I hope you'll join me. This is my show. [Music]
People Analytics is one of the hottest topics there is in HR at the moment. A data revolution is starting to make companies radically rethink their approaches to attracting, retaining and developing talent. Technology is transforming the kind of qualitative research that underpins employer branding and employee engagement strategies, providing the ability to generate instant insights that would have previously taken months of work to produce. My guest this week is Andrew Marritt from Organization View. Andrew is a pioneer in the field of text analytics for HR and has some fascinating insights into the potential for data in the talent space. • In the interview we discuss: • The relevance of Big Data for HR • The power of using technology to bring meaning to the free text data found in performance reviews and employee surveys on a massive scale • How free text analytics can help to develop subtle differences in employer brand messaging to give maximum appeal across multiple target audiences • The potential for real time analysis of job applications to generate automated smart follow up questions, particularly in the graduate recruitment space Andrew also share his thoughts on the application of text analytics to email and workplace collaborations systems to spot conversational trends within organizations Subscribe to this podcast in iTunes