The Bold Blueprint Podcast
The Bold Blueprint Avideh Zakhor Positive, supportive individuals will
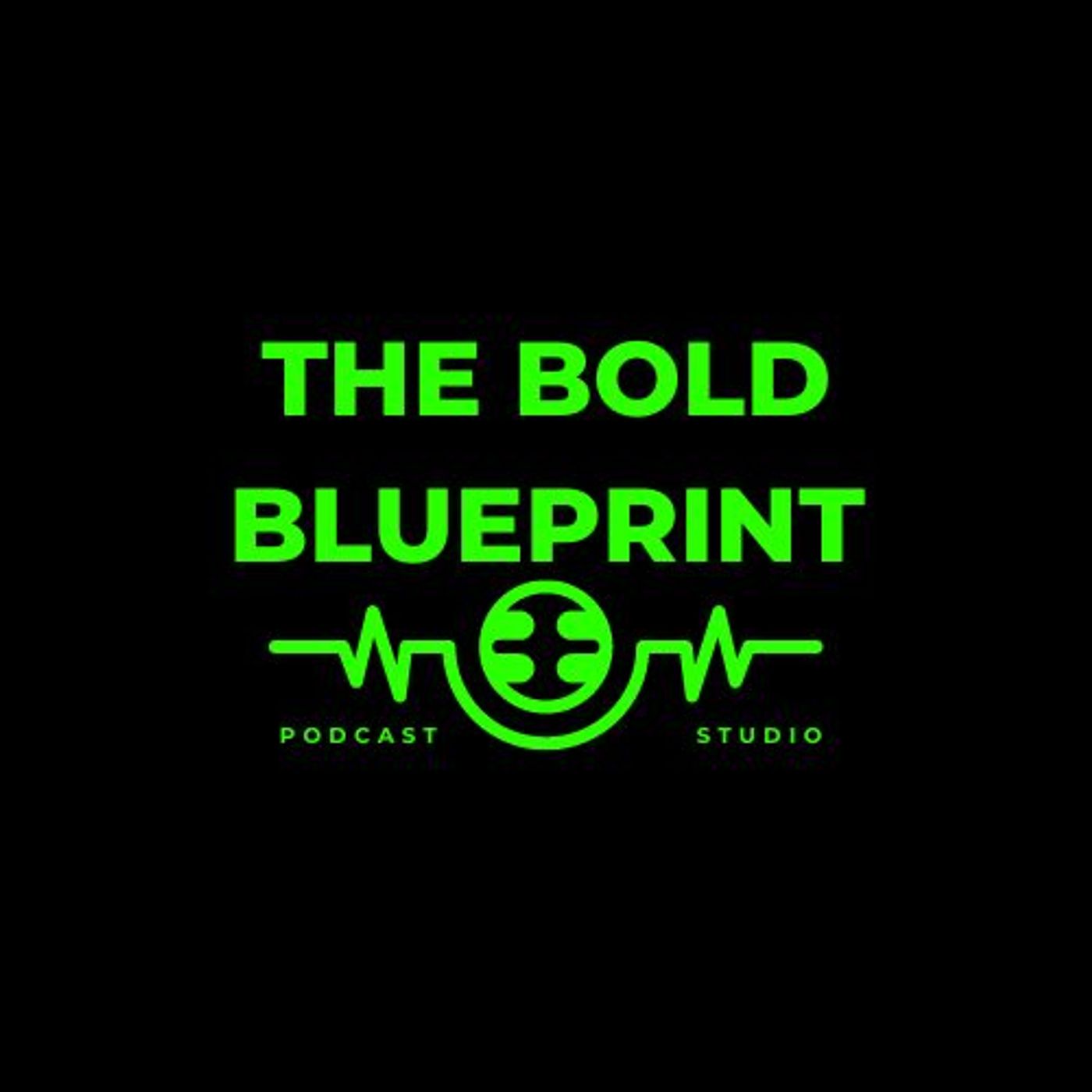
Hey Amazon Prime members, why pay more for groceries when you can save big on thousands of items at Amazon Fresh? Shop Prime exclusive deals and save up to 50% on weekly grocery favorites. Plus save 10% on Amazon brands like our new brand Amazon Saver, 365 by Whole Foods Market, Aplenty and more. Come back for new deals rotating every week. Don't miss out on savings. Shop Prime exclusive deals at Amazon Fresh. Select varieties. Bada. Bada Boom. Sold. Huh? Just sold my car on Carvana. Dropping it off and getting paid today. Oh ready? What? You still haven't sold yours? You told me about it months ago. I just... Is the offer good? Oh, it offers great. Don't have another car yet? I could trade it in for this car I love. Come on! What are we waiting for? You're right. Let's go! Whether you're looking to sell your car right now or just whenever feels right, go to Carvana.com and sell your car the convenient way. Terms and conditions apply. Just a few announcements today. One is that your proposals are due. The second one is I will lead them and try to give you feedback by next week and actually it will help if you go to your email. Actually I have your email at this one the first day of the class so that's not a big deal. I might respond to you by email about questions. This is not clear so if you do get it please try to respond. If not, I'll just come back with written comments on this by next week. The second announcement is the GSR position open. Pick up the handout on the way out in my lab and the DMS class. And then the third announcement is that there's a lab homework to next week. I think that's one to the last lab homework. There's one more lab on restoration and that's about it. For those of you who might still be unsettled about what you want to do for your term project, come back and see me, come back and see me for additional suggestions. It might be probably too late but there might be, there could be other topics that I could propose to you that could excite you more. In particular I had talked about this earlier in the semester but I forgot to put it in the handout that I gave you. If you're not excited about what you're doing and you want to do something on multi-camera sensor network type stuff, come see me I can propose some papers to read and possible topics and projects to were considered in that area. Just so that I have, I think there's nothing to hang this on, okay. Just so that I have an idea how many people are doing group projects. Okay and are you two in the same group? Okay and you had to, you had Mary also in your same as one. Okay so just about everybody else is doing independent projects, is that right? Wow. Okay and just another quick show of how many people chose topics from the handout they gave or my suggestions. One, two, three, four and just really quickly, let me just go around David, what did you pick? Okay, excellent. Oh, okay. Okay and Howard, you are Howard, right? Okay, okay. So in any event of the four of you who picked from the list that I gave, in case you're not terribly excited by content retrieval then you want to do something on camera networks and sensor networks stuff like that, come see me at the end of the class. Okay any other, any questions or comments? If I get started. Great, so what we're going to talk about today is we're going to skip over chapter four almost and jump right into chapter five. There's only one topic in chapter four that's interesting is homeomorphic signal process, homeomorphic processing. I will get to that in the middle of the restoration stuff, not today, but we're going to start looking at restoration. [silence] [silence] [silence] [silence] Okay, so what I wanted to do on chapter four, I mean basically chapter four talks about the effects of high pass profiling, which we all know mostly about it, but there's really one interesting figure in it that I'd like to just quickly go over and, that is figure, find it, it's quicker to find it here than the other. 11 and 12, 4 11 and 4 12. The reason I want to talk about it now, not that it has anything specifically to do with enhancement, but when we get to the compression part of the class, knowing this or having seen this is kind of useful and interesting. So this, if you can switch to the, and so I'll just spend five minutes on showing this concentration of energy in the Fourier domain type idea and then launch on image restoration immediately after that. So what you see on the left is that famous same image that this guy Gonzales on Woods is using as his test image and when you, you know, it's got the A's that get increase in size, it's got these random patches, squares, lines, etc. And then it has the Fourier transform of it in this domain. And this image is 500 pixels by 500 pixels and Gonzales rather than talking about, for example, in his frequency domain, the axes instead of going from minus pi to pi in both directions like we were normally used to, he kind of, he kind of, if the image is 500 by 500, he talks about a 500 by 500 pixel frequency domain as well. So if you read the, read the caption and don't understand, don't worry about it too much. So, he's superimposed a bunch of circles with different radii. One, two, three, four, five. The first circle is circle of radius 5, then 15, then 30, then this is 80, and then the last one is 230. So from here, in his, in his world, from here to here is 250, from here to here is 500, the whole line is 500, right? And then, and this is 500 pixels as well. So this is a circle of radius 230, this has got to be 80, and this has got to be 30, and then 15, and 5, you probably can't see. But the point that we want to show is, you know, if you keep those coefficients that are within those circles and throw away everything else, what do you get? Well, you get this. So, and this is working its right backwards. So this is, if, this is the resolving images, if you keep everything inside the circle of radius 230, as you can see, it's, it's almost indistinguishable from the original, and you've only thrown away half a percent of the energy of the, of the four-year domain. You move one to the left, this is the reconstructed image, if you kept the coefficients, your DFT coefficients essentially, inside the circle of radius 80. And if you do that, you've only thrown away 2% of the energy. This is the circle of radius 30, and you can see that visually, now you've gotten quite a bit of, and a ringing going on. And at this point, with the circle of radius 30, you have thrown away only 3. Hey Amazon Prime members, why pay more for groceries when you can save big on thousands of items at Amazon Fresh? Shop Prime exclusive deals and save up to 50% on weekly grocery favorites. Plus save 10% on Amazon brands, like our new brand Amazon Saver, 365 by Whole Foods Market, Aplenty, and more. Come back for new deals rotating every week. Don't miss out on savings. Shop Prime exclusive deals at Amazon Fresh. Select varieties. We wear our work, day by day, stitch by stitch. At Dickies, we believe work is what we're made of. So, whether you're gearing up for a new project, or looking to add some tried and true work wear to your collection. Remember, the Dickies has been standing the test of time for a reason. The work wear isn't just about looking good. It's about performing under pressure and lasting through the toughest jobs. Head over to Dickies.com and use the promo code Workwear20 at Checkout to save 20% on your purchase. It's the perfect time to experience the quality and reliability that has made Dickies a trusted name for over a century. 0.6% of the energy in the in the in the in the in the Fourier domain. And finally, when you get to here, let's say this is a circle of radius 5. And at this point, but by keeping everything inside a circle of radius 5, the image is entirely on in what's the word for that. Not legible, but unrecognizable. However, from an energy point of view, you you again only thrown away 8% of the energy. So, why am I showing this? Well, it's probably, you could probably come out with a better example where, for example, at this point, we don't have so much ringing artifact. But, and and there are ways of doing that. But, but the point is that most of the energy in the Fourier domain is concentrated in low frequency, particularly for natural imagery. This is not a natural image. This is something that this guy made of himself with these patches and the A's and stuff like that. But if you had a more natural looking imagery, even all the way to this point, and I think I've shown that in Jay Len's book all the way to this point, where you, where you have a circle of radius 5 or the radius 10 or something like that, you still have most of the legibility of the of the picture. You can recognize it quite, quite easily. And, and this is the property that's used in, in the, in for compression, where in that DFT and DCT have compaction properties that have few coefficients contain most of the energy in the Fourier domain. And if you just keep those until 90% or 95% of other coefficients to zero, then you, you can do, you can reconstruct the signal almost in a good way. And as we'll, we'll get back to Fourier domain, you don't necessarily have to, this, this is called zone coding, where you predefined the zone and then keep all the coefficients inside that, throw away everything outside it. Another way of doing it is just threshold the coefficients and say, look, all the coefficients below this level are going to get thrown away. And if you do that, then obviously you get a much better reconstruction. And that's called threshold coding. That's really all I want to show in these pictures at this point. I'm going to now move on to chapter five. Actually, let me just show one more thing. Yeah, and that's figure 424. Let's see. Where's figure 424? Here we go. Okay, so suffice it to say that if you do, if you start with that same A picture, if you do high pass filtering, these are kind of the kinds of, the kinds of kind of images and signals that you would get. High pass filtering has the property that if, because the DC value of the filter at DC is zero, then the resulting image, on average, have DC value of zero. That means if I add up all the pixels, I'm going to get zero. This is why it's tremendously dark. So in case, I can't remember whether in any of your homeworks, you've done any high pass filtering examples, but in case you haven't, roughly speaking visually, this is what a high pass filter looks like. And as we go from here to here, the radius of the high pass filter keeps increasing. And generally, people use high pass filtering as a way kind of to sharpen images. And one of the byproducts of high pass filtering that you should be aware of is ringing artifacts, which should be apparent in A here, but frankly, it's very good in the book. It's not even on my computer screen, and I thought if it comes back on your television screen, there's a whole lot of ringing in this picture that's visible in the book, but obviously not visible here. So that's one of the drawbacks of high pass filtering, is that you should be generally aware of. Okay, that's it. Move on to restoration. And off to chapter five. Okay, so we'll come back to this screen in just a second, but let me talk about why we do restoration and why it is different from enhancement. So generally speaking, as I talked about earlier, enhancement is the process where you start with an image and it's kind of a subjective process. You just want it to look better. Okay, so enhancement is this image should look better, and this look is kind of subjective. Okay, now it is true, as I said, if you if you're making the image look better so that a computer vision application can do better, then you can come up with quantitative way of improving it. But generally speaking, it's it's you wanted to the human eye to look nicer. So it's kind of an improvement or subjective improvement of of the quality of the image. When we deal with restoration, the two actually come close in certain instances, but when you deal with restoration, what has happened is something specifically bad has happened to a good image. So some noise has been added, some motion blur has corrupted your image, some other thing some other bad thing has happened to your image. So so image has been degraded essentially by something. And it could be by noise, it could be some blur, it could be that some it has gone through the signal has gone through a system that you didn't want it to go through, like for example atmospheric turbulence. So generally speaking when you deal with restoration, you you have much more objective models rather than having subjective models. And you use criteriums like error like mean square error in order to to do this. So what do I mean by something some degradation has happened to your signal? Well, here's an example if you can roll up just a little bit. So you start with your good old image that you would have liked to obtain and it goes through some degradation box. Let's say could this system could be a linear shifting variant system with impulse response H over N1 and N2 and outcomes. And that's not the only bad thing that happens to it. We also add some noise to it. And what comes out is what we observe. So let's say we would send the Hubble telescope up up in the space and it's sending these pictures home and we know that there's something wrong with these pictures. We know that the telescope is out of focus and there's too much noise and all those other things. And the question is observing the noisy degraded version can be reconstructed to recover F. So in this case G of X comma Y is H convolved with F plus some noise. So it would be H of X comma Y convolved with F of X comma Y plus A to of X comma Y where this is the noise component. And by the way sometimes things are so degraded that you can't really recover the original signal. In the case of the Hubble telescope they through the images around the scientific community, the image processing community on Earth. And it turned out that the support of this LSI filter that had corrupted the images was so large it was something like 40 pixels by 40 pixels that nobody could actually make him look any better. And the final fix was to send these these manned guys to the Hubble and change the lens manually. A human had to go up there and fix the system, fix the optical system. So that's that's a bad publicity for image processing field but it's the truth. So it's the truth but at the same time it's good because we can go ask for. Hey Amazon Prime members, why pay more for groceries when you can save big on thousands of items at Amazon Fresh? Shop Prime exclusive deals and save up to 50% on weekly grocery favorites. Plus save 10% on Amazon brands like our new brand Amazon Saver. 365 by Whole Foods market, a plenty and more. Come back for new deals rotating every week. Don't miss out on savings. Shop Prime exclusive deals at Amazon Fresh. Select varieties. We wear our work day by day, stitch by stitch. At Dickies we believe work is what we're made of. So whether you're gearing up for a new project or looking to add some tried and true work wear to your collection. Remember the Dickies has been standing the test of time for a reason. The work wear isn't just about looking good. It's about performing under pressure and lasting through the toughest jobs. Head over to Dickies.com and use the promo code workwear20 at checkout to save 20% on your purchase. It's the perfect time to experience the quality and reliability that has made Dickies a trusted name for over a century. We're funding to help us solve those problems. So next time we don't have to send a human to fix the problem, right? Okay, you can fill up and in academics too long, right? So how does the restoration process work where you start with G and then you do something to it? This is the restoration box. Okay, it could be linear timing variant thing or it could be nonlinear and outcomes f hat of x comma y. And then the goal is to make sure this guy f hat looks as much as possible like this guy f. So the goal would be to minimize, for example, the expected value of f of x comma y minus f hat of x comma y, the whole thing squared. Okay, and so this is, so this is kind of the big picture as to what's happening. And there could be occasions where there's no degradation by linear shifting variant system and the only noise is added or there could be a situation where there's no noise and this is added. So you consider all of those things at the same time. So for the rest of this lecture for today, I'm just going to assume that h, this filter here, is identity. That means the only corruption is noise. Okay. And so the problem that we have is we observe G of x comma y, which is f plus noise. This is the original image that we're interested in. This is noise. And this is the observed. And we're trying to come up with a way of getting that this guy f back. Okay. And so before I dive deeply into the various techniques that we use to do that, I want to, I want to make few assumptions. One of them is that we're going to assume that this noise is independent of special coordinates. So in other words, we don't have extra noise in the middle than the sides or in the sides of the middle with any of those things. And also it's uncorrelated with respect to the image itself. In other words, it's, this is a big assumption. It doesn't always happen in practice. In other words, you don't get extra noise, for example, in the bright parts of the image rather than the dark parts or the edge parts rather than the texture parts or et cetera, et cetera. So the amount of noise that's been added is kind of independent of what's going on in the signal itself. The signal and noise are uncorrelated. Actually, I'd like to take a quick show of hand. Now, how many of you are taking 225A or know about wiener filtering in a formal way? Oh, okay. So not everybody. Okay. So that's the topic for next time's lecture, but that would help me adjust the level of it. I was initially just going to go right through it very fast five minutes, but I don't think I should do that. So here's the different kinds of noise that one might consider in practice. What's the one that we always consider in signal processing courses? And in real life and everything? Gaussian, exactly. And the probability density of a Gaussian random variable is one over a square root of two pi sigma and then e to the minus z minus mu squared over two pi sigma squared. Okay, where the variance is sigma squared and mean is just mu and the shape of it is like this. And what can we say about where does Gaussian noise pop up in practice? And why do we use Gaussian noise in all the time? Well, Gaussian noise comes up in, you can model very nicely electronic noise. It can also model noise due to sensor noise, do for example, to poor elimination or maybe high temperature, etc. And one of the reasons Gaussian noise is due so frequently is because what? Amaletically it's very easy to handle, right? It has mathematical properties that suppose that so many times in situations where you even don't have a clue what you're going to be like. You assume Gaussian so you can analyze the situation so that you can reach a conclusion assuming Gaussian this is the best filter I have to come out with. Because if you assume something more complicated, let's say like Erlang or Gamma noise or something like that, the analysis would just get crippled and it can't make any headways. So you can develop some intuition by assuming something that's slightly off or not exactly 100% correct or maybe it could be correct, maybe not, but we don't know. But we have an answer for it. Okay, so Gaussian noise is excellent for that kind of analysis. And some of a bunch of random variables, sometimes Gaussian is referred to as normal distribution. And the reason normal distribution is important is if you add a bunch of random variables of almost any distribution, the law of large number tells you that it approximates Gaussian. Most notably, this class size is too small, but almost every semester I teach large courses like 60 and over students, the distribution of the grade is almost all the time Gaussian. It is, it just happens, add the height, you add up the height of the people and large number of the other people's weights, all of those things. The Gaussian occurs in practice a lot. Except that you know in real life Gaussian mathematically has a tail that goes on all the way to minus infinity to plus infinity, but like adding up average people's heights and weights, it really doesn't go to infinity. Like maybe the fattest person on earth is about a thousand pounds or something. But it's not infinity. No 300, 400, I can't, it's 600. Is that right? Alright, but it's, I mean the point is it's not infinity, right? It doesn't really go all the way. And then the same thing with heights, you know, seven foot, eight foot and that's about it. Don't get that. And just, just so that you know when you talk, when you talk about Gaussian and you talk about what percentage of the population is one sigma a way. But you go talk to your, your doctor about your kids height and weight, you know, he says, your kid is in, I don't know, X percentile, height wise and Y percentile. But if the distribution is Gaussian, then 70% of the values are within, it's called one sigma. And what sigma is the standard deviation. So that means it's between the range mu minus sigma to mu plus sigma. And you can say 95% is within two sigma. Okay. And so the, the, the, the casual saying is if you want to say somebody's really smart, you say, oh, this guy is 6th of my way. That means that he's smarter than 90, I forgot 99.9 and a huge number of 9s. Or, or it could be a negative thing. So this guy is 6 sigma bad or something. Okay. Any questions on this? All right. So the next distribution we talk about is Raleigh, or Rayleigh. And not the bicycle, the distribution. And the mathematical expression for that is z over b times z minus a e to the minus z minus a squared over b. This is for z larger than a and it's 0 for z smaller than a. So the mean for this mu is a plus squared of b pi over 4. And the variance is b times 4 minus pi over 4. And this is typically used for range imaging. This is, this represents noise in range imaging applications. The next distribution, for the rest of this course, we won't do much beyond using Gaussian and uniform, but I just wanted to throw these at you and show it to you just for sake of completeness anyway. If you can roll up so people can see the top half of that. Great. So the next distribution is Erlang. Hey Amazon Prime members, why pay more for groceries when you can save big on thousands of items at Amazon Fresh? Shop prime exclusive deals and save up to 50% on weekly grocery favorites. Plus save 10% on Amazon brands, like our new brand Amazon Saver. 365 by Whole Foods market, a plenty and more. Come back for new deals rotating every week. Don't miss out on savings. Shop prime exclusive deals at Amazon Fresh. Select varieties. We wear our work day by day, stitch by stitch. At Dickies, we believe work is what we're made of. So whether you're gearing up for a new project or looking to add some tried and true work wear to your collection, remember that Dickies has been standing the test of time for a reason. Their work wear isn't just about looking good. It's about performing under pressure and lasting through the toughest jobs. Head over to Dickies.com and use the promo code workwear20 at checkout to save 20% on your purchase. It's the perfect time to experience the quality and reliability that has made Dickies a trusted name for over a century. Or gamma, noise, and in this case P of Z is A to the B, Z to the B minus 1, E to the minus A, Z over B minus 1 factorial for Z larger than 0. And 0 for Z smaller than 0. And this is to sometimes it's referred to as gamma noise, sometimes it's rare length and it's used, it models the noise and laser imaging. The mean for it is B over A and the variance is B over A squared. And the fourth one is exponential, which is a special case of gamma or Erlang. And here P of Z is A times E to the minus A, Z, Z larger than 0. And what's, when you take probability, what's one distribution that's exponential? What's the famous processing probability? Poisson, that's right. And what is it in Poisson process that has exponential distribution? Exactly, in an arrival time between the packets. So that's the thing that's exponential. And what field uses this Poisson process a lot to model things, to draw conclusions, etc. Networking. For the longest time, people in networking community used to think that the inner or what times within the packets on the internet are Poisson. And then finally, I forgot, it was 1992. And the name of the guy escaped my life. Some guy had Bell Labs and his name was stars with E.L. L. something. Not El-Kawi. Can't remember his name. Anyway, the guys at Bell Labs came up with this idea is that they actually, I mean he used one place that could have done it. Why? Because Bell Labs was tied to the telephone company and they could do a lot of measurement based stuff. So they put probes and they analyze, I don't know, a month force of data coming out of, in and out of AT&T's networks at some location. And they plotted it and they examined it and they found out that absolutely, positively the Poisson assumption was wrong. And in fact, the traffic has a lot of what's called long tail, as well as self-similarity patterns in it. It has a fractal type behavior. So right around '92, God, I have to remember the guy's name. He became very famous as a result of this piece of work. And of course, as we speak, not Bell Labs doesn't exist anymore, right? It got split into Lucent versus the other, the AT&T. And AT&T just got merged with SBC. And I'm sure that guy is not a researcher at AT&T anymore. But that's a, that's a different point. In any event, so this, this, this, this gets used a lot in networking, as well as in laser imaging applications as well. And finally, I mean, for our, our class is not on networking, it's on image processing, but the noise in laser imaging can be modeled as, as, as exponential. And finally, the last one I want to talk about is uniform. That doesn't happen too often in practice. P(z) is 1 over b minus a for z between b and a, and it's 0 otherwise. And in this case, the mean is a plus b over 2, and the variance is a b minus a squared over 12. Okay? Not many noises in nature perform in a uniform way. But if you wanted to, if you wanted to write software and you wanted to generate random number generators, you use uniform distribution a lot. Also, also analytically, it's very simple to keep track of, just like Gaussian is. I would still think Gaussian is, has nicer properties because the, if you have a Gaussian random process, then you can show that the power spectrum of it is also Gaussian and it's got nice, the Fourier transform of the Gaussian is also a Gaussian. So Gaussian is fantastic from an analytical handling kind of point of view. And the last kind of noise that is important in image processing, and I probably shouldn't have put it last, is impulse noise. Or sometimes they call it salt and pepper noise. Okay? This happens when there's like a quick transient, okay? Such as, you know, faulty switching or something like that in the image acquisition process. And the probability of z, the random variable of z, is P sub a, if z is equal to a, P sub b, this is P sub b, if z is equal to b, and it's zero otherwise. Okay? So if, for example, if b is larger than a, then the, the b guys will, will show up as dots, as, as light lights, light dots. And if, otherwise, and then, sorry, in this case, b is, is a, is a light light, then a will show up as a dark dot. And I'll show you examples of this. And just one second. Okay. Okay. So what you have there, and figure 5.2, is this various probability distribution. So on the upper left here, you've got Gaussian. This is mu, mu minus sigma, mu plus sigma. So this is the one sigma region of Gaussian or normal distribution. And it used to be that if you bought CRC books, the whole back of it was basically telling you, if you're X sigma away, what's the area under the curve? So this used to be good, get quantized and published in CRC books. And I bet you can now find the same things on the internet. This is Raleigh. So it's zero all the way to A, and then it kind of dies down like this. This is gamma or Erlang. This is exponential. Actually, one other distribution, other that I think about it that we didn't talk about, but gets used a lot in image compression is what? The DC coefficients of DCT doesn't have a Gaussian distribution. It has a Laplacean distribution. So I'll talk about it when we get too much compression. This is a uniform distribution. So it's between A and B, 1 over B minus A. So the area under it is 1. And this is the impulse, or salt and pepper. So in this case, A is smaller than B. So with probability P sub A, we've got A, probability P sub B, we've got B. And so if, for example, P sub A is equal to zero, you end up giving what this, this is, or P sub B is equal to zero, then it's called unipolar. But the case of P sub A and P sub B are not equal to zero, this is called bipolar. But that's minor detail. The truth is, if P sub A and P sub B are non-zero and they're roughly the same magnitude, you end up getting what's called salt and pepper-looking images. So here's a picture of a circle instead of a square instead of another square, and we add different kinds of noises to it. And this is shown here. And now, again, Gonzales is a big fan of histograms. So he's plotting, after he adds noises of various levels, he plots the histogram of the resulting image. So the reason he had, the reason he has dark black gray and slightly lighter is because then he wants to have a tri-modal histogram. The bump here corresponds to the black, the bump in the middle corresponds to this square that's grayish here, and the third bump corresponds to this circle. So then he adds a Gaussian noise, and so synthetically to this image, and then he plots a histogram, as you would expect. You see that you get three bumps, and the shape of these bumps are Gaussian. Then he does the same thing with Raleigh, and you can see that if you go back to the distribution of Raleigh, it kind of starts here, it goes up, and then has a long tail to the right, and you can see the same thing here. And that's not surprising, right? Gamma, more or less the same thing, exponential, looks pretty exponential, uniform. So now you have the three bumps, but each one has looks flat on top, and then finally. Hey Amazon Prime members, why pay more for groceries when you can save big on thousands of items at Amazon Fresh? Shop prime exclusive deals and save up to 50% on weekly grocery favorites. Plus save 10% on Amazon brands, like our new brand Amazon Saver, 365 by Whole Foods Market, Aplenty, and more. Come back for new deals rotating every week. Don't miss out on savings. Shop prime exclusive deals at Amazon Fresh. Select varieties. We wear our work, day by day, stitch by stitch. At Dickies, we believe work is what we're made of. So whether you're gearing up for a new project, or looking to add some tried and true work wear to your collection, remember that Dickies has been standing the test of time for a reason. The work wear isn't just about looking good. It's about performing under pressure and lasting through the toughest jobs. Head over to Dickies.com and use the promo code Workwear20 at checkout to save 20% on your purchase. It's the perfect time to experience the quality and reliability that has made Dickies a trusted name for over a century. Okay, the salt and pepper noise. And salt and pepper noise, I have to bring it to your attention. Actually, it's not even on my screen, so it's definitely not in your screen. There's a bump here. There's a bump here. And then there's a large, long thin line here, white line. And there's another long thin line noticeable here. If you're looking at your book and figure 5.4 D, or the last, sorry, L, you can see this white thing here and this. And then what are those? That's the, this is the pepper noise and this is the salt noise. Okay. It is this, it's salt noise means, this is the salt and pepper picture. So salt means you have white dots, these white guys, okay, that are appearing here. And yeah. And the pepper noise is the black dots here. So the question becomes, how to, how to, what are the techniques we can use to remove this kind of noise? And of course, it's, it's different if you, if your noise is, you have to use a different technique if your, if your signal, if your noise is Gaussian, then it is salt and pepper. As we'll see in just a second, low pass filtering does well for Gaussian type signals, Gaussian type noises, whereas for salt and pepper noises, we have to use other techniques like, like what? Anyone? I think we talked about that a little bit last time previously. Exactly. Nonlinear operators like median, min, max, that kind of a thing. And that's kind of what today's lecture is all about. Now before I dive into the various filters we can use to, to get rid of these kinds of noises, I'd like to just briefly talk about the situation when you have a periodic noise. So here's a picture I think of, of the image that was corrupted by a sinusoidal noise. And the easiest way, if you have this periodic kind of noise, then the chances are you go, you're going to get spikes in the frequency domain. So for example, this is conjugate conjugate symmetric portion of that, this is the same as that, this and this are paradox, and this and this are paradox. So if you look at the frequency domain and then remove those, you can easily remove the screening artifact that's visible. Unfortunately, Gonzales doesn't have the processed version of this. He might have it at a later point in the book, but we'll probably show that in a little bit when we get to the latter part of this chapter. Okay? So let me, then start talking about, let's come back to the papers. Let me talk about the various filtering techniques that we can use to get rid of these, these nasty noises. So if you come back to the paper, please, we, we want to talk about mean filters as our first class. Okay? So the first thing that we want to consider is arithmetic mean, where we look at the local neighborhood around each pixel to do the computation that's needed to replace the value of that pixel. Not that the window doesn't, you don't look at the window and then we place that window with the new window, but rather you look at the window around the pixel just to remove the, just to change or process the, the center of the window. So our window is, as the same notation as before, s of x comma y, it's m by n, and this is our window. And our processing scheme is to say, for, for mean filter is f hat of x and y is just one over m n, summed over all the pixels of the, the corrupted signal, g of s t, where s and t are members of s of x, y. So you look at all the pixels inside the window, you add them up together, average them, and that's the answer. It's just plain old low pass filtering. Okay? And then the second filter we, we deal with is geometric mean. In this case, f hat of x comma y is just the geometric means of the pixels within a window. The product of all the g of s comma t's for all the s and t's that are in the window, that are member of s x y. It's a lot of words for something very simple, okay? And then the third kind of thing that we will consider is counter harmonic. Actually, let me start with harmonic and initial counter harmonic. So then we have the harmonic mean filter, which is given by f hat of x comma y. The process version is m n over the summation of s and t again in s x y of one over g of s comma t. And then finally, the counter harmonic, where f hat of x comma y is given by the ratio of two things, the summation of g of s and t to the power of q plus one over the summation of g of s comma t to the power of q. And this is again, this is again for all the s and t's are in s x y, s and t's are in s x y. So to note here is that if q is larger than zero, then this counter harmonic guy removes pepper noise. And if q is smaller than zero, it removes salt noise, okay? And this geometric mean guy up here, it's kind of similar, it does has similar effects to the arithmetic mean, but you lose less detail. So it's similar to arithmetic, but lose less detail. And finally, this harmonic filter that works well for salt noise, but fails for pepper. And you can see why, because it's a special case of this counter harmonic filter, right? It's the case where the q is smaller than zero. These two things become related to each other, and so that's where the characteristic comes from. So in order to show these things in action, let me switch back to the monitor and look at figure 5.7, 5.8, and 5.9. So what you have there on the upper left is an original x-ray image. I believe it's a printed circuit board of some kind. That's up here. And on the right-hand side, you have the same image, but with additive Gaussian noise. And just intuitively, before we move on, if you have additive Gaussian noise, what would you expect would remove that the best? Some sort of low-pass filtering, right? So needless to say, you apply a 3x3 average and kind of filter arithmetic mean to this signal, and you get this signal down here. And again, I don't know how many of you have your books, but it doesn't even show up on my screen, so I'm pretty sure it doesn't show on the TV screen. By the time you apply the 3x3, you remove some of the noise, right? But what else have you done? You've also blurred your image. Hey Amazon Prime members, why pay more for groceries when you can save big on thousands of items at Amazon Fresh? Shop Prime exclusive deals and save up to 50% on weekly grocery favorites. Plus, save 10% on Amazon brands, like our new brand Amazon Saver, 365 by Whole Foods Market, a plenty and more. Come back for new deals rotating every week. Don't miss out on savings. Shop Prime exclusive deals at Amazon Fresh. Select varieties. We wear our work, day by day, stitch by stitch. At Dickies, we believe work is what we're made of. So, whether you're gearing up for a new project, or looking to add some tried and true work wear to your collection, remember that Dickies has been standing the test of time for a reason. Their work wear isn't just about looking good. It's about performing under pressure and last thing through the toughest jobs. Head over to Dickies.com and use the promo code Workwear20 at checkout to save 20% on your purchase. It's the perfect time to experience the quality and reliability that is made Dickies a trusted name for over a century. And this is kind of applying the geometric mean filter. Again, it does a fairly good job of removing the noise. In particular, if you are looking at your book, you can see that a lot of the noise in the constant intensity regions here have been removed by the time you hear. But this is a little bit more detailed than their asthmatic mean. So, geometric mean filter is slightly better in terms of not losing the sharpness of the original image. Now, let's move on and let's add salt and pepper noise. So, this is the original. This is with additive Gaussian noise and this is salt and pepper noise. And there's a lot of it that has been added. Sorry, this is the pepper noise. This is not salt and pepper. This is just this original signal but corrupted by pepper noise. So, all you see is a bunch of black dots on top of the original, on top of this guy. And this is the same thing but with a bunch of white dots, salt noise added on top. And this is what you get if you apply the contraharmonic filter of order 1.5 and this is contraharmonic filter of order -1.5. So, what do you expect to get? Well, when Q, just like we talked about here, when Q is positive, we remove all the pepper noise and you still see a little bit of white salt noises remaining. And when Q is negative, which is in this case, you've removed some of the salt noise but some of the pepper noise is still evident. Actually, I'm seeing kind of the, let me look at it truly. Does this come across on the screen at all? I don't think so. Yeah, I should be honest with you. I'm looking at the very, I'm looking at the very pictures in the book itself. And for example, in D, in part D, where it's supposed to have removed the salt noise and left on pepper noise, I don't quite see that as dramatically. Well, yeah, I don't quite see that as dramatically as I should. It doesn't really matter because most of the time when you, the bottom line is that this contraharmonic filter is not a very good thing to use because first of all, you have both salt and pepper noise, it can't handle both of them at the same time. And as you'll see in just five minutes, the, oh, oh, I know, okay, okay, sorry, I know why I was confused, scratch all of what I said. This one, we're not starting with the picture that has both salt and pepper on top of each other. We have one corrupted image with just pepper, one corrupted image just with salt, and we apply the 1.5 order, which is supposed to remove pepper noise to this, and we apply Q equals minus 1.5 to this. Okay, that's, so there's no, for a minute, I thought I'm talking about something else. So, I'm not supposed to see pepper noise at all in this. We didn't start with the thing that has salt and pepper, we just applied one filter to this, the right filter to this, and the right filter to that. And it's done a fairly good job of removing the white dots here and the black dots here. But these are two different filters applied to two different images. And if you now go down here and you reverse, you apply the Q larger than zero to this one, and Q smaller than zero to this one, this is the output that you get, and as you can see, it's garbled. So, the point is with contour harmonic filters, you have to look at the value of Q, and if you're removing salt noise, you've got to apply something, Q smaller than zero, if you're applying pepper noise, something smaller. That's all there is to it. And that's exactly, so it's good when you have either salt or pepper noise. But if you've got both of them together, then you have to move on to order statistic type filters or nonlinear filters like median, max, min, et cetera. So let's move on to that, order statistic filter. So we've got median again, where you look at a small window, Sxy, find the median, and then replace the middle pixel, replace the x comma y with the median value. And the next one is the max filter, f hat of x comma y, is just the max of g of s comma t, and in the region Sxy. And then you've got the min filter, which is just the same thing, f of x comma y, is just the mean of Sxy g of s comma t. And finally, we have the midpoint, where you go with the half of maximum plus minimum. And the beauty of median filter now is that it can handle images that have both salt and pepper noise added on top of each other. But what's the drawback of it? Becomes clear when we talk about adaptive techniques, but what's the drawback of it? What if even in window that you're looking, there's so much salt and pepper noise that by accident, the median is one of the salt and pepper noise values, then you're kind of in trouble. But for the most part, median filter does a fairly good job. So here is an image, now we're on track. Here's an image that's been corrupted with both, let's switch back to the computer. Hello. Here's an image that's been corrupted with both salt and pepper noise on the upper left here. And to the right of it is the result of a three by three median filter. And as you can see, most of the salt and pepper noise has been removed. If you're looking at your book, you can see that there's few more random stuff, black and white ones still remain. For example, if you look at the book, if you have your book, there's a black dot here that's remaining, there's a couple of black dots here, etc. So what do you do? You can apply median filter on top of this, the process median signal. And you get this and that pretty much removes everything. And then if you apply median filter again, on top of this, you get this signal. And actually, there's a theorem that coil at Purdue came up with many years ago, I would say in mid-80s. And it goes like this. Under certain conditions, if you keep applying the median filter on top of the signal over and over again, after a while, it converges to a signal and it won't change anymore. And they call that signal the root signal. So the median filtering, it's advantageous many times to keep applying it kind of over and over. And as you can see, one of the good things about median filtering is that it doesn't blur out your signal. And why is that good? Well, images have edges and having sharp images is always useful. So it's important to end up having images that look nice and short. So now let's move and talk about max and min filter. So this is the same corrupted signal here, where we apply the on the left, where we apply. Hey Amazon Prime members, why pay more for groceries when you can save big on thousands of items at Amazon Fresh? Shop Prime exclusive deals and save up to 50% on weekly grocery favorites. Plus save 10% on Amazon brands, like our new brand Amazon Saver, 365 by Whole Foods Market, a plenty and more. Come back for new deals rotating every week. Don't miss out on savings. Shop Prime exclusive deals at Amazon Fresh. Select varieties. We wear our work, day by day, stitch by stitch. At Dickies, we believe work is what we're made of. So whether you're gearing up for a new project, or looking to add some tried and true work wear to your collection, remember that Dickies has been standing the test of time for a reason. The work wear isn't just about looking good. It's about performing under pressure and lasting through the toughest jobs. Head over to Dickies.com and use the promo code WorkWear20 at checkout to save 20% on your purchase. It's the perfect time to experience the quality and reliability that has made Dickies a trusted name for over a century. The Max Filter. And what do you expect the Max Filter would do? It's a 3x3 Max Filter. And just so that you can compare, the original looks like this. This is the original we started off with. Look at the thickness of these black lines here. And again, I don't know how well it shows up on your computer screen. Okay. And now we apply the noisy version and then we apply the Max Filter. What happened? Yeah. Some of the dark pixels have been removed. The thickness of these these things are a lot. Look at the teeth here, the parallel black lines. Have a mental picture of the thickness of each line and then go back compared to this. You can see they're a lot thicker here than they are in the other place. Maybe it's even true of these, but let me keep that in mind. Not so much of these guys, but it's quite noticeable here. So the Max Filter, along with removing salt and pepper noises, also removes some of the dark pixels. Okay. So also removes some dark pixels. And the min filter accidentally also removes some white pixels. So if I go back to the computer monitor to the right, you can see that the white dots that were surrounding here, you see these dots here, these white dots here, they're all gone when you apply the min filter here. And does that make sense? Yeah, because when you apply the min filter, you're looking at the window, you're always picking the minimum. So when you pick the minimum, yeah, sure, you get rid of all the white pixels because whites are not have high large intensity values. So everything you do, it's almost like taking drugs. You take a drug, it fixes something, but it always messes up something else. The same thing with these filters is you want to call with a filter that he moves the noise, but doesn't attach the signal. The drug for cancer attacked the tumor, but leave all the healthy cells kind of intact. Okay. And the last filter I want to talk about is, and show some pictures, is alpha-trained mean filter. Okay. And it's kind of a complicated filter, but what it does is it allows us to both remove, depending upon how you pick the parameters in it, it allows us both to remove in one shot, glossion noises, as well as salt and pepper noise. So let me write down the expression for that if you can come back on paper. So we're talking about alpha-trimmed mean filter. Okay. So in this case, f hat of x comma y is 1 over mn minus d, and remember, the window size was m by n times mn minus d, sorry, times the summation over all the pixels s and t that are in the window are not g of x comma y, but g sub r. So there's a, I'd like to emphasize that r if I can open this set of, here we go. So there's an r here. Okay. And what is it? Well, what do we mean by g sub r of s and t? It's all the pixels in g of s and t, excluding d over two widest gray levels and d over two darkest gray levels. So you kind of look at your window, let's say d is equal to 2. So d over 2 is 1. You look at your window and you say, okay, these are all the gray levels that I have in my window. Let's say, this is my window. In this case is 4 by 4, and it's got 5, 4, 3, 2, 1, etc, etc. You write down the gray levels from the smallest to the largest, kind of like you do with median. When you do median, you're kind of trying to find the intensity value for which half the pixels are below half the pixels above. Here, you're removing kind of the outliers. You're removing d over 2 brightest pixels and d over 2 darkest pixels. And then you do the averaging. So you can see why it's good for both getting rid of Gaussian noise and it kind of does median filtering. If there was salt and pepper noise, you're hoping that the salt and pepper noise will be the two ends. And you're removing those intensity values before you compute the average so that the averaging is not corrupted. Because you see, if you apply average and kind of filter to a salt and pepper noise signal, it doesn't really work well. And I'll show that to you in just a second. So by removing these extreme values, kind of the outliers, then you give yourself a chance of removing the Gaussian noise. Because now you're averaging all the other pixels that had the Gaussian noise, but in the process of doing that computation, you've removed the salt and pepper values from your local window. And so a good example of how all these schemes work is in this picture 512, if you can switch to the computer. So what we have is the original image that is then corrupted by additive uniform noise. And then on top of that, we add salt and pepper noise on top of this. So this signal has additive noise, and this this signal has additive noise plus salt and pepper noise. And then so now we apply this 5x5 filters in order to try to fix this image. And if you apply a 5x5 arithmetic mean filter, just an averaging type of filter, this is what you get. And you can see this looks terrible. It's still full of noise, full of garbage, full of everything else. This is if you apply geometric mean filter, also disaster. Not much of an improvement over that. This is if you apply median filter. The median filter has done a fairly good job of removing the salt and pepper noise, but the uniform additive noise is still there. And this is what happens if you apply the alpha trim. And actually, I don't expect you will see much difference on the television screen there. But in the book, if you look at 512 between E and F, for those of you who have the book with you, you can see the difference between them. Actually, let me try a little experiment. Can you focus? Can you focus on these two pictures? Down, down, down. As much as you possibly can. Okay. Yeah, I don't think it... Let me come all over here to see, to experience what you are, I don't think you see any difference between these two. I don't think it comes out on the screen. Does it? No. I don't think so. But if you look at your... If you have the book in front of you, and I encourage you to do that. Actually, I switched to one of the main reasons I switched to Gonzalez and Woods, by the way, was because they had the pictures electronically online. But little did I realize that there's no LCD projector in this room. And we're still going through the projection system on the TV, which kind of distorts or low-pass filters. It just blurs out a lot of the things that's going... On the screen, actually, it's almost as good as the print. I think he's done a fairly good job of that. Trying to think. Yeah. I mean, I could turn that screen around, but I don't think people all the way in the back of the class could see that. The other thing I could do is I could investigate just bringing my own portable LCD projector to the class. But I don't think that's terribly easy. Let me investigate that a little bit. It's almost a moot point. I mean, I switched from limb to this because I got the pictures now electronically, and I thought now people can actually see the difference. And we're kind of back to square one. But anyway, the point here is that coming back to these things is that the arithmetic mean, the geometric mean, they're not going to do very well because you've got salt and pepper noise. The median filter gets through the salt and pepper noise. The alpha-treme min filter does slightly better in terms of median filter in that it's kind of removed both the salt and pepper noise. And it's addressed a little bit of the additive uniform noise. Let me wrap up today's lecture by talking about one last topic in the next five minutes. And that has to do with adaptive local noise reduction. And as you would expect, this is nothing but a adaptive kind of local technique. So basically what you say is how I process my image of the particular point, x, y, has to do with the local statistics surrounding that pixels. Has to do with the local mean and the local variance surrounding that pixel. So it'll be f of f hat of x comma y, the process to signal is the given the observed g of x comma y minus sigma squared n, sigma squared l of g of x comma y minus m sub l. And m sub l is the local mean which is given by one over mn summation of g of x comma y with all the x and y's in s x y. Sorry, s comma t, s comma t. And then sigma squared l, I'm not going to write this down, but it's local variance. We already talked about it last time. And sigma squared n is the noise variance. And in looking at this and this kind of a situation, right off the bat, what's the problem with having an approach like this? That's correct. How do you know the noise? And in particular, if the noise variance varies across your image, there's more noise in this here than it's there, etc., estimating the noise variance locally is terribly difficult. So I mean, what do you do in practice? Oh, let's just assume noise variance is the same all across the image. Good. Nevertheless, how do you compute the noise variance all across the image? And that's not very easy. So first, let me convince you that this thing roughly speaking, even if we let's forget about the problem of not knowing the noise variance, let's for me, let's just convince you this is a good approach to begin with. And then we add just the problem of how do you compute noise variance. So why would this approach work? Well, if sigma squared n is much, much smaller than sigma squared l, that means the amount of noise in a region is much smaller than the variance, right? Then ideally, what would you like to get? Well, if the noise is small and sigma squared l is the variance of the signal, that means there's a lot of activity going on edges and textures and stuff like that, you don't want to touch the signal. There's no noise. You want to pass the signal right through. So in that case, this thing becomes close to zero and f hat becomes approximately equal to g. So good. That's desirable. What if sigma squared n is much, much larger than sigma squared l? Well, then this term becomes big. It could even become like if three times bigger or four times bigger, then it could be the whole value of f hat could become negative. That's a disaster. We don't want that to happen. So if this happens, you want to make sure or doesn't have to be much, much bigger. Even if it's just bigger than this, then what you want to do is just approximate, let's say, sigma squared n over sigma squared l to be approximately equal to one. Don't let it, in other words, even if, even if for some reason, I mean, if we already know, there's a whole lot of ambiguity and uncertainty and errors and inaccuracies in estimating sigma squared n, the variance of noise. And if after you do that, with all that uncertainty, the number comes out bigger than the local variance, for God's sake, don't generate negative pixel values. This is just going to kill you. So in that case, just make the assumption that that sigma squared n is approximately equal to sigma squared l. In that case, this ratio becomes one. And what are we doing? Then the signal, in that case, then f hat becomes approximately g minus g plus ml. So we replace the value of the center pixel with the local mean. And does that make sense? Yeah. When there's a lot of noise, don't average out the pixels. Don't let that center pixel go through. Average out the noise around you with the hopes that that averaging process got rid of the noise and the signal got through. So intuitively, this is kind of how you do it. And you look at your, one possible way to estimate your sigma squared n, if you've got an image, just for example, go to the local, to go to a piece of the image visually where the, you know the intensity is flat. I measure the noise, measure the variance there. And that gives you an idea what sigma squared n is. So you have to be kind of creative as to how you estimate the noise variance. So what you see here is the result of of the variance techniques applied to the original signal. I won't show the, well let me just quickly go back to the original and show it to you. This is the original, just refresh kind of your mind with it. And here's what, what you get if you add additive Gaussian noise, zero mean variance a thousand. Here's what you get if you do just arithmetic mean filtering, just average out seven by seven pixels, terribly blurred. This is what you get if you do geometric mean, a little bit less blurred but still quite noisy and these lines have become very, very thick. And this is what you get if you do adaptive noise reduction where you're computing locally, what's what's happening in your neighborhood. And you either, you either, if sigma squared n is smaller than L, then you compute using this, this equation we have. If sigma squared n is larger than L, sigma squared L, you just approximate it to be equal to one. And, and if you come back here to the, to the picture, you can see that this signal is quite a bit better. So the, the thing I want you to walk away from is you got Gaussian noise, you got to do linear filtering of some kind, adaptive, this, that, and the other. Got salt and pepper noise, you got to do either median filtering or the next technique I'll talk about that on Wednesday of next week, which is this adaptive median filter which can handle situations where you have both Gaussian noise and salt and pepper noise. So I'll see you all on Wednesday. And if you guys can remember to bring your book, that would be great because this system is really not working. I, I see the image is fantastic on the screen here and I'm pretty sure you don't see anything down there. And don't forget to pick up your, your graded homework Hey Amazon Prime members, why pay more for groceries when you can save big on thousands of items at Amazon Fresh? Shop Prime exclusive deals and save up to 50% on weekly grocery favorites. Plus save 10% on Amazon brands, like our new brand Amazon Saver, 365 by Whole Foods Market, Aplenty, and more. Come back for new deals rotating every week. Don't miss out on savings. Shop Prime exclusive deals at Amazon Fresh. Select varieties. We wear our work day by day, stitch by stitch. At Dickies, we believe work is what we're made of. So whether you're gearing up for a new project or looking to add some tried and true work wear to your collection, remember that Dickies has been standing the test of time for a reason. Their work wear isn't just about looking good. It's about performing under pressure and lasting through the toughest jobs. Head over to Dickies.com and use the promo code Workwear20 at checkout to save 20% on your purchase. It's the perfect time to experience the quality and reliability that has made Dickies a trusted name for over a century.