The Bold Blueprint Podcast
The Bold Blueprint Avideh Zakhor Instead of seeing difficulties as roadblocks
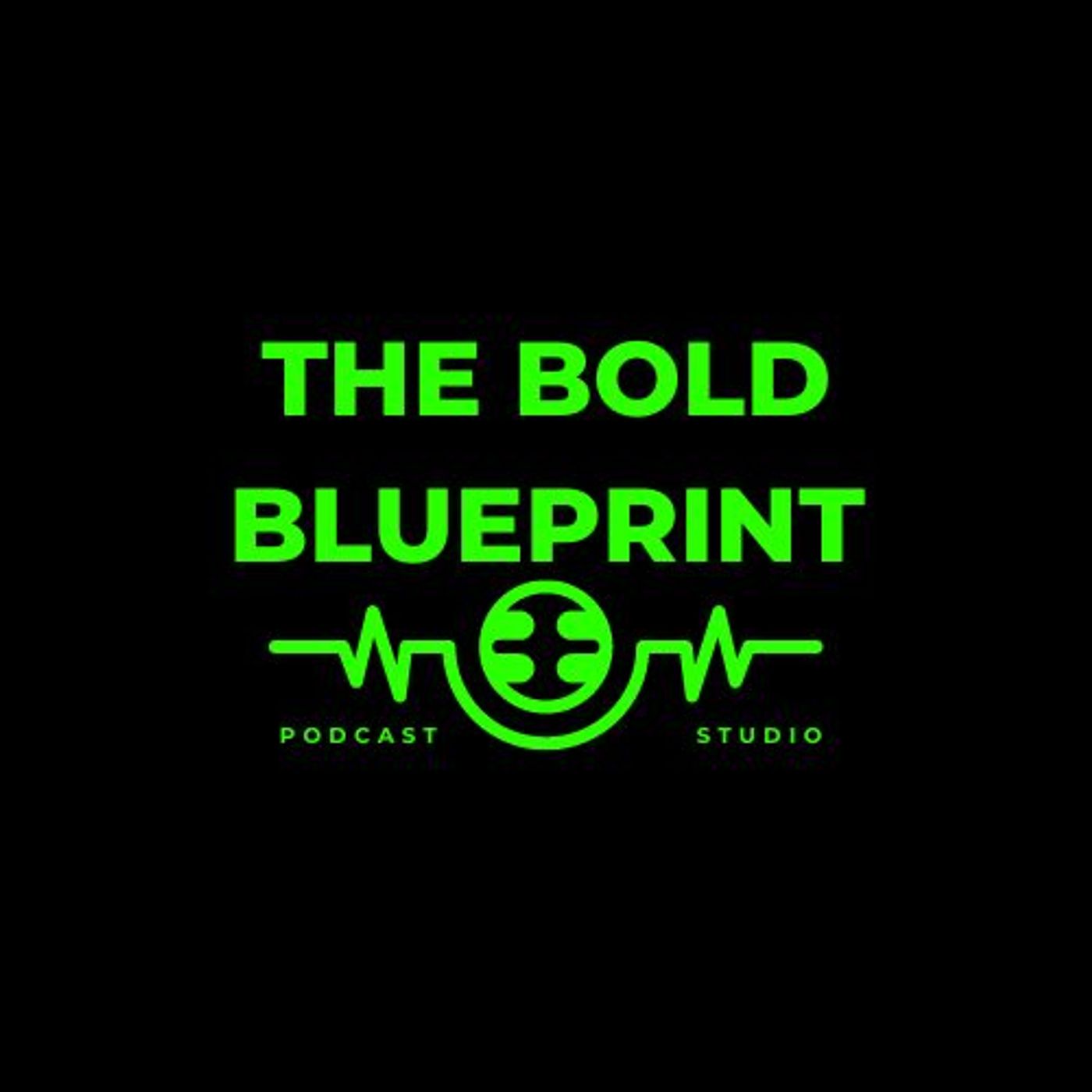
Hey Amazon Prime members, why pay more for groceries when you can save big on thousands of items at Amazon Fresh? Shop Prime exclusive deals and save up to 50% on weekly grocery favorites. Plus save 10% on Amazon brands like our new brand Amazon Saver, 365 by Whole Foods Market, Aplenty and more. Come back for new deals rotating every week. Don't miss out on savings. Shop Prime exclusive deals at Amazon Fresh. Select varieties. We wear our work day by day, stitch by stitch. At Dickies, we believe work is what we're made of. So whether you're gearing up for a new project or looking to add some tried and true work wear to your collection, remember that Dickies has been standing the test of time for a reason. Their work wear isn't just about looking good. It's about performing under pressure and lasting through the toughest jobs. Head over to Dickies.com and use the promo code workwear20 at checkout to save 20% on your purchase. It's the perfect time to experience the quality and reliability that has made Dickies a trusted name for over a century. So this is a homework due today. Make sure you hand in your homework right here. Pick up the solution. I need to fire this up before I can you please topple this. Okay. It's the best way to pop some discs while you're bending over pulling something. Thank you. Actually, can you put it one level lower so I can see people just put it at that. Thank you. Why? Am I covering it? Yeah. It's really heavy, these monitors. Oh, this thing is already on. Okay, very good. Okay, I don't have to spend a lot of time logging in. So hand in the homework, pick up the solution, pick up your grade at homework. homework number six is on the web. And as a as a hand as a special homework, it's not off of the Jay Lim's book. It's a actual design of 2D filters using the transformation technique. Are there any questions or comments? Okay. So what we're going to talk about today is image enhancement. That is the topic. And roughly speaking, that corresponds to chapter three in Gonzalez and Woods, and it corresponds to chapter eight in Jay Lim's book. So let me fire up my various power points here. Don't do that to me. Okay, I'm going to have to make some changes here. Okay. Unknown. How could it be unknown? I put the material on just so that you know on my web page, but there's a step I forgot to do. And that is CH mod user other groups plus RWX. In other words, change the mode so that it's accessible. So I forgot to do that one step. And now I'm trying to use SSH to log in. Cindy, can you perhaps come here? Maybe you can shine some. I'm using remote SSH to log in. It says the host is unknown. Why would it be unknown? I do that all the time for my office. You hit connect. It says the host is unknown. Should I try to connect to a different location? What do you tell me what you want to do? Ah, let's kill it and start it again. Let's just quick... apologies. Hey Amazon Prime members. Why pay more for groceries when you can save big on thousands of items at Amazon Fresh? Shop Prime exclusive deals and save up to 50% on weekly grocery favorites. Plus save 10% on Amazon brands, like our new brand Amazon Saver, 365 by Whole Foods Market, Uplenty and more. Come back for new deals rotating every week. Don't miss out on savings. Shop Prime exclusive deals at Amazon Fresh. Select varieties. We wear our work day by day, stitch by stitch. At Dickies, we believe work is what we're made of. So, whether you're gearing up for a new project or looking to add some tried and true work wear to your collection. Remember that Dickies has been standing the test of time for a reason. The work wear isn't just about looking good. It's about performing under pressure and lasting through the toughest jobs. Head over to Dickies.com and use the promo code Workwear20 at checkout to save 20% on your purchase. It's the perfect time to experience the quality and reliability that has made Dickies a trusted name for over a century. Actually, you know what? We can just directly get the artwork for Gonzalez and Wood off its webpage. Do you know where it is? Yeah, let's just try one more time. And if this doesn't work, we'll just do that. Why is it going to end this to us? Let's try to see, you know. Am I mistyping something? Well, let me just, here's the thing. Let me just log out and I have log in, log off presentation and I'll re-log in. Okay. Yeah, that's unbelievable. So, I can just get going on the lecture and when we get to the, I mean, there's another backup. I can just show the pictures off here, but it's much, of course, it's much nicer to show the power, the electronic version. Okay. So, I see that we have a symphony of coughing people. I might just join you myself in a few minutes. So, what we're going to talk about today is image enhancement. And what do I mean by image enhancement? Well, the main task when you're doing enhancement is try to come up with an enhanced version of an image so that you can see things better or I'll log in and it'll take five minutes before it gets all my stuff going there. So, the main reason you want to do enhancement is because you want to bring out something in the image that wasn't visible before. And as I said last time, many times the human observer or the human user is the ultimate judge of the final outcome of the enhancement. And so, at the end of the day, you know, whether the enhancement was successful or not is very, very much a subjective thing. I mean, does this image not look better than before we processed it? Subjecting is not there. Sometimes that's not the case and that the actual, you have an actual objective in mind. You're enhancing an image so that your part recognition software can recognize different parts in a factory or an automation process or something. In that case, you don't really, you can come up with objective functions. For example, you can say, "Look, my recognition rate went up by 10% because I enhanced my image because I pre-processed it before I do the actual recognition." So, there's some tasks where you can actually quantitatively measure the improvement that enhancement brought about other tasks. Other situations, it's the human observer that's the ultimate judge. So, let me get going on image enhancements today and we will give this one last try. And if it doesn't work, we will use an entirely different option of just getting chapter three pictures off the web. Well, fine-setting password. It just doesn't recognize this thing. Okay, I think Cindy, we ought to find, I think what we do is just go to Gonzalez and Woods. You guys, you go to the Gonzalez Woods webpage at all. Okay, so here's how you find it. You go to Google and then you type Gonzalez Woods. I'm tired of all this. Okay, web page for that and there is the, let's see, teaching students faculty and then instructor's manual PowerPoint presentation. Oh, I hope I don't have to download the whole thing. Oh, no, I don't. It's good. So, what we want is chapter three artwork. Oh, a lot of hardware. [ Laughter ] Okay, bye now. I don't want to do the entire registration. I use evaluation. Very good. PowerPoint. Okay. Hey Amazon Prime members, why pay more for groceries when you can save big on thousands of items at Amazon Fresh? Shop Prime exclusive deals and save up to 50% on weekly grocery favorites. Plus, save 10% on Amazon Brands, like our new brand Amazon Saver, 365 by Whole Foods Market, a plenty and more. Come back for new deals rotating every week. Don't miss out on savings. Shop Prime exclusive deals at Amazon Fresh. Select varieties. We wear our work day by day, stitch by stitch. At Dickies, we believe work is what we're made of. So, whether you're gearing up for a new project or looking to add some tried and true workware to your collection, remember that Dickies has been standing the test of time for a reason. The workware isn't just about looking good. It's about performing under pressure and lasting through the toughest jobs. Head over to Dickies.com and use the promo code Workware20 at checkout to save 20% on your purchase. It's the perfect time to experience the quality and reliability that is made Dickies a trusted name for over a century. So, let me just fire up one more PowerPoint that I had put on my... What happened? They lost again? Right. My son grieved over that all last night. Okay. So, now... There's one more PowerPoint with nice pictures I want to show. Okay. No, not that one. Jesus Christ. Okay. Put that down. Okay. So, we'll come back to those. We're all set. We've recovered from the CH mod problem. Okay. So, image enhancement. So, if you can come back to my writing here, we can actually do a normal lecture today. So, the purpose is to modify an image to bring out features that were hidden otherwise, hidden otherwise. Okay. And it could be the final user of this thing can be either humans or it could be machine. Okay. And roughly speaking, we're going to talk about two classes of image enhancements. One of them is spatial domain. The other one is frequency domain. Spatial domain means that you're actually operating on the pixels and that's the main topic of today's lecture and Wednesday's lecture. And frequency domain is that you've converted it into the Fourier domain and you do operations there. Okay. So, the main topic that we will talk about today, well, we don't have the fan anymore to blow the pictures. Good. I mean, the papers. Okay. So, what we're going to talk about today is spatial filtering. All right. And, or spatial domain filtering. And the basic idea is in a spatial domain process, you start with an image f of x comma y. This is the input that's the given. And g of x comma y is the enhanced image, the output. And what you do is a space domain process in general would be shown by a transformation T. So, you apply transformation T to this input image f of x comma y and what comes out is g of x comma y. Okay. And there's many kinds of transformation. This transformation, for a given output point, for example, it could be a function of location x comma y. The output location x comma y can be just a function of that pixels at x comma y or it could be a function of a neighborhood of that pixel. Okay. So T can be either can be either only a function of x comma y pixels in f or the neighboring pixels in f in x comma y around the neighboring pixels around x comma y but in f. Okay. So, we're going to consider both cases. The simplest case that if you can roll up, please. So, the simplest case that we're going to consider is where the size of T is just one by one. Okay. That means that T looks at the single pixel in f of x comma y in order to determine the value of g at location x comma y. It doesn't look at the neighborhood. So, it's a good time now to actually show this picture from Gonzales and Woods to elaborate that point if you can switch back to the to the computer here. Hello. Great. So. So, if this is the, I think that Chris is just rolling on the floor. Tell me why are you rolling on the floor. Hello, hello. Right. We have to do that occasionally. To the camera person. Okay. So, here's the here's the image and this is the origin x and y. And so, this just just simply shows that if you could you could look at location x comma y to come out to figure out what g is that that value can look at the neighborhood of it. So, that's that's pretty straightforward. So, if you're just coming back here onto the paper down here. So, if I'm writing down for the case where s is just for the case, we're looking at one by one pixel, then you can write s equals t of r where s is the output pixel at a particular location and r is just the input pixel. Okay. Output pixel at x comma y. And this is the input pixel at x comma y. And some of the simplest cases of this function transformation of s transformation t applied to the input pixel running r where you only operate on one pixel is shown in this picture here. Where this axis along the horizontal axis is r, the vertical axis is s as is a transform of r and the shape of the transformations of this form. And so, essentially, what is this what this is doing? It's it's increasing the the it's increasing the the contrast of the image that that you're dealing with. And you can make the this sigmoid type of function even sharper. And what kind of operation does this this transformation correspond to? Hey Amazon Prime members, why pay more for groceries when you can save big on thousands of items at Amazon Fresh shop prime exclusive deals and save up to 50% on weekly grocery favorites plus save 10% on Amazon brands like our new brand Amazon Saver 365 by Whole Foods Market a plenty and more come back for new deals rotating every week. Don't miss out on savings shop prime exclusive deals at Amazon Fresh select varieties. We wear our work day by day stitch by stitch. At Dickies, we believe work is what we're made of. So, whether you're gearing up for a new project or looking to add some tried and true work where to your collection, remember that Dickies has been standing the test of time for a reason. Their work where isn't just about looking good. It's about performing under pressure and lasting through the toughest jobs. Head over to Dickies.com and use the promo code work where 20 at checkout to save 20% on your purchase. It's the perfect time to experience the quality and reliability that has made Dickies a trusted name for over a century. Thresholding right all the input values that are below intensity m come out as dark as zero and all the intensity values larger than m end up being just the maximum value. So, it's kind of like thresholding the image at a certain level m everything above m is white everything below m is black. So, this is picture 3.2 of Gonzalez and Woods. And instead of having a one by one transformation, as I mentioned earlier, you could have a small mask, for example, a three by three region. So, more complex T. You could have a three by three region around surrounding a pixel that allows you to do the transformation. And these are typically called it could be three by three or five by five or seven by seven. And these are generally called masks. And here are, and these guys, the simple case that are typically referred to as gray level transformation. So, we'll come to this more complex one again in next next time's lecture. But for now, I'm going to concentrate on gray level transformations. So, what are some, what are some typical possibilities of a gray level transformation that's shown in this picture here? Okay. On the, on the horizontal axis, you have the input gray level R in the, on the vertical axis, you have output gray level S. And what these various curves show is possible transformations, possible transformations between input and output. So, let me start with the simplest of all of them, which is the identity, a straight line going from the diagonal. The input range is between zero and L minus one, the output range is between zero and L minus one, just have a straight line. Another simple straight line type thing is the, is the negative transformation, where it goes from diagonally, but from top left to the lower right. And what that does is generates the negative of an image. And I'll show you examples of that in just a second. Another interesting one is a log transformation. And the log transformation is, is used to compress, it essentially what it does is it maps a small range of the input gray levels to a large range of the output gray levels. And I'll show you examples of that. And, and the other, the, the, and then, besides the log, you have the end truth. And then, and the other side, you have inverse log and the end power. So these are power transformations. And as, I'll, I'll, I'll go through examples of each one of these transformation and to give you some intuition of what these things do, to, to actual images. So, coming back here, let me start by our transformation that's called image negative. Okay. So here, s is equal to l minus one minus r. That's the straight line coming down this way. This is the negative. But this is r, and this is s, goes 0, l to minus one, 0 to l minus one. Okay. And this is also shown in figure 3.3 of Gonzales and Woods. So an example of, of an image, so you might want to do the negative transformation in order to make some feature come up better. So here's an example of a mammogram on the left is the original. And what you do is you just invert it. And you can see the various dark regions a lot better than you would have seen in this, in this region. And whether or not this was successful enhancement strictly depends upon the application you're in and what the medical, medical personnel who were working with this think about it. Coming back to the picture, to the paper here, another transformation we're going to consider is log transformation. And here we have s is equal to some constant log of one plus r. Okay. And the shape of this, this, this curve is something of this form. This is r, this is s, and it looks concave looking like that. Okay. So what does this transformation do? Well, inherently what it's going to do is going to expand the values of dark pixels. You can, I've drawn this a little bit too conservatively. So let me redraw this. This is the log transformation. So these are the dark pixels here and a small range of pixels here get mapped to a wide range of pixels there. Okay. So what, on average, what do you expect this transformation to do to your image? Does it make it darker or lighter? Right. So it expands, that's correct. Expand values of dark pixels and therefore it's going to make the overall picture somewhat lighter. Okay. It also, what else does the log function do? It compresses kind of the dynamic range of the image. So let me show an example of application of this log transformation, not to a natural image, but to actually a different image. What do you think this thing is? I'm showing you. Go back to the PC, please. That's correct. On the left, what you see is a four-year spectrum of some image. Actually, I forgot what image it is. And the ratio between the DC coefficients, the low frequency coefficient and the AC coefficients is so huge that it's beyond the dynamic range of most displays. So if you look at the four-year transform or the spectrum on a linear scale, this is what it looks like. And on the other hand, if you took a log of this thing, then you can actually see a lot more details that were invisible here. You can see there's almost a straight line going that way. There's a bit of a straight line going this way. There's a circular pattern going here. So essentially, what log is doing is it's reducing the dynamic range so you can actually see. So in the intensity domain, the values of your four-year transform went from, I don't know, a million to one, the ratio between the smallest and the largest, after you take log, then the log of one is zero, log of a million, it's six. So you've reduced the dynamic range by taking log and you can see a lot more variation into your image. Okay, moving on. So let me come back to the paper again. So the log transformation has the thing that it changes the dynamic range or compresses the dynamic range. And what other situation do we use the log transformation in image coding or audio coding? In audio coding, there's a thing called mu-log coding. How many of you have heard of it or know about it? We don't have many audio people here. So what happens is that when you start with the audio signal, again, the dynamic range is so high that the best way to compress it is to apply a log transformation to it so that you've reduced the dynamic range so that then you can quantize and do other things. So we'll talk about that later on in the course when we talk about image and video compression and audio compression, etc. Okay, the next transformation I want to talk about coming back to the paper down here is a power law transformation. Okay, so in this case S, the output is some constant R to the power of gamma, okay, where C and gamma are positive quantities. And to show that phenomena, I'm going to go here. So show figure 3.6 in themselves on words. And what you've got here is input level R, output level S, and if gamma is equal to one, once again, you get the identity transformation, no changes. The values of gamma smaller than one, the curves look this way and the values of gamma larger than one, the curves look this way. So for gamma, for example, in this range, for smaller values of gamma, it could look a little bit like log. So the power law transformation is a lot more powerful than log because log is just more in curve here by adjusting gamma. You can have any shape curve that you want to do. And once again, what is the property of this kind of transformation? Well, when the input level, when gamma is larger than 2.5, a large values of input gets transformed into a small range of output gray. So the images tend to become a little bit darker. On the other hand, when gamma is smaller than one, the images kind of tend to be a little bit lighter. Now, the power law transformation is quite important because there's a process called gamma correction that uses this power law transformation. How many of you have heard of gamma correction? Good. So what does gamma correction mean? Let me just throw it at you. You just heard of the computers. That's exactly what it's used actually. So here's what happens. By the time you're doing image processing, you've got an array of numbers, f of x, comma y. It's a two-dimensional array of numbers. And the real question is, how were those numbers obtained, a, and b, if you want to visualize those numbers on a output device. The output device could be on a computer screen. He was not talking about it. It could be a cathode ray tube or it could be on television screen or it could be on a printer. So there are all these devices that enable us to look at images. Now, each and every one of those devices have a different characteristic. And I have a different input output characteristic. So what do I mean by that? For example, suppose that if you can look back at the, at the paper here, suppose I'm dealing with a CRT, a cathode ray tube. What can I say for cathode ray tube, there's a characteristic. In other words, there's the input voltage you apply to it. And then there's luminance that you get out of it. Luminance i. And for now, let's just not worry about the color part, the RGB, et cetera. Just, just pure black and white type of a situation. We get a luminance i. Ideally, and so this input voltage is supposed to do your f of x, comma y is the input image that you have, et cetera. Ideally, what would you like to get? What you would like to get is just a straight line going this way. You like, as the input increases, you want the luminance to increase as well. Kind of like A to D's. When people design A to D's, they want to have a straight line staircase as an input output. Input is so much voltage, the output is this much voltage, et cetera. But in real life, it looks something like this. i is equal to u to the power of gamma. Where gamma for cathode ray tubes is somewhere in the neighborhood of 2.5 or something like that. Okay. So, what happens is that... Hey Amazon Prime members, why pay more for groceries when you can save big on thousands of items at Amazon Fresh? Shop Prime exclusive deals and save up to 50% on weekly grocery favorites. Plus, save 10% on Amazon brands, like our new brand Amazon Saver, 365 by Whole Foods Market, a plenty and more. Come back for new deals rotating every week. Don't miss out on savings. Shop Prime exclusive deals at Amazon Fresh. Select varieties. We wear our work day by day, stitch by stitch. At Dickies, we believe work is what we're made of. So, whether you're gearing up for a new project or looking to add some tried and true work wear to your collection, remember that Dickies has been standing the test of time for a reason. Their work wear isn't just about looking good. It's about performing under pressure and lasting through the toughest jobs. Head over to Dickies.com and use the promo code Workwear20 at checkout to save 20% on your purchase. It's the perfect time to experience the quality and reliability that has made Dickies a trusted name for over a century. So, the image that... So, if you have an f of x comma y and you want it to display it on its CRT, then if you just supply it as is, the image is going to tend to look what? A little bit too dark or a little bit too bright. A little bit too dark. And this example is shown right here. So, here you have a linear scale kind of a image. The intensity is decreasing or increasing linearly from left to right. This is what you want to show on the monitor. And if you just send it to the monitor that has this kind of characteristic, the UI characteristic here, it comes out a little bit darker. So, what you do is you pre-compensate or pre-correct it ahead of time by undoing this transformation here to generate something that's a little bit lighter than the original so that after it goes through the monitor function, which does this, what comes out is what you had in mind already. So, this is called gamma correction. So, the idea of correcting the input ahead of time according to some power law, kind of a function, correcting the input image according to some power law so that it's rendered correctly in the output device. It's called gamma correction. And so, every cathode ray tube has a different kind of correction. The flat screen looks different. It's different from the old CRTs. And actually, technically, I don't think you call this cathode ray tube at all. There's no rays here, right? Cathode ray tube means there's a ray tube and it shoots electrons and there's all kinds of radiations and all that, right? This is not cathode ray tube at all. I forgot the technology they use. Do we have a display of people in this room? You might not send the LCD, right? I forgot that. So, that's LCD. So, anyway, this monitor has a different input output character, luminance versus input voltage characteristic. It has a different characteristic from a CRT. It has a different characteristic from a printer. And so, one of the problems that people face is on the internet, when you put up an image on your web page, you want it to be done in such a way that it looks good on as many computers as possible. It looks good on this, looks good on the CRTs. And so, you have to do some kind of an averaging to make sure that on average it looks good. In general, also, when you have a picture on your computer and you want to print it, you also have to do an order of gamma correction so that it looks nice on your printer. You don't want it to look too washed out and you don't want it to look too dark on your printer. So, there's another set of between rendering devices. You have to keep doing gamma correction so that the image comes out just right on the, on the, on different rendering devices. Is that, is that clear, everyone? And I just, I just can't resist, but now, but, but show this, this picture in Giro's presentation. Right. So, so what I talked about, it talks a little bit about this gamma correction stuff and it has some nice slides that, that I like to, to show it to you guys. Is this big enough or you want me to make it bigger? 122. I'm going to make it 150. Is that better? Okay. So, what we have here, essentially, he's asking you, can you see the circle? Actually, I could see it on this monitor, but by the time it's gone through, do those images. And can you see the circle? Yeah. That's, that's the thing we talked about last time, which was called what? Weber's law. Right. So, here we have a background intensity i and you increase it by delta i and you ask yourself, but what, what percentage of y should I increase delta i so that it's noticeable? And the answer is somewhere in the range of 1% to 2% is, is what you see. Right. And we all, it's called Weber's fraction of Weber's law, whatever you want to call it. And that's called the visibility threshold. Now, suppose you come back and you say, okay, now I'm going to stack up my intensity values. I have a, I have, I want to have 255 or 256 because that corresponds to 8 bits. Distinct intensity levels so that I can tell the difference from this level to the next level. Okay. So, and so, so for example, if the intensity at, at, at, at, at the lowest intensity level is i mean, the next one is i mean times 1.02. The next one is the previous one times another 1.02. The next one is the previous one times another 1.02. And I stack, I do this operation 255 times. It's like compounding interest kind of a thing, right? And what value do I get from my i max? Well, you get 1.02 to the power of 255 times i mean. So in other words, the ratio between i max and i mean is this expression or the constant is 0.02 or 0.01 depending on whatever you think the Weber's fraction is, right? And if you plug more 0.01 or 0.02, let's say if you plug 0.01, then the number that you get i max over i mean ends up being of the order of 13. And if you plug 0.02, the number you end up getting is roughly 156. That means the, the luminance value between maximum and minimum, the ratio between those is somewhere between 13 and 156. And that's about right because the typical display contrast for a cathode ray tube is 100 to 1. And for printing on paper is something like 10 to 1, okay? And it's, it's, it's this kind of an expression that suggests or encourages us to do uniform quantization in the law of intensity domain rather than intensity itself. Because if you do it on the intensity itself, the ratio between max and min is extremely high. And you're going to lose a lot of bits because that ratio is high. On the other hand, if you took the log, then you can, you've compressed the range and you can do much nicer. So the next slide is, is the gamma correction slide, which we talked about a little bit, but I like to show it. So for a cathode ray tube, the u versus, so the horizontal axis is voltage. Do you guys want me to make it bigger on your screen? Can you read that? Let's try one, one 75. One 75. Okay, that's slightly better. Can you read that now? Yeah. Okay, so the horizontal axis is voltage u, which represents level f of an image and vertical luminance. So i is a proctomically proportional to u to the power of gamma and for the CRT, gamma is a bit somewhere between 2, 2.3, etc. And so many times, the cameras contain gamma pre-distortion circuit to undo this thing so that when it comes out, it looks just right. And so if I now reverse these pictures, if I now, for example, instead of plotting u as a function of i as a function of u, I reverse this and plot u as a function of i. What I get is u as a function of i, I get i to the power of 1 over gamma is equal to u, which is this blue curve. And for all intents and purposes, people approximate this curve with the log curve, even though they're not exactly the same. Many times, the pre-distortion, you simply approximate this i to the power of 1 over gamma with the log curve. We already show that curve is that obeyed by that. I think that's all I want to say. This goes on talking about a lot of other other stuff that I don't think I want to talk about at this point. But I thought since there were nice power points, we might as well talk about them. So we already talked about this pre-compensation of pre-distortion, and that's bigger 3.7 of Gonzales and Woods. Okay, so let's look at some examples of applying the power law transformation to some actual images coming back here. That's actually a picture of an MRI of a spine. I just saw a doctor yesterday where I showed my MRI of this kind of spine actually. I didn't have a fracture, thank god, but I had like many bulging discs, C4, C5, L4, L4, all over this disc coming out. But yeah, it makes you wonder like why are they not staying? So here is the original image that comes right out of it. And by the way, for those of you who have gone, how many people have gone to an MRI process? Oh, a lot of you. Yeah, you walk out of the room. I mean, first you're sitting inside that tube, which is extremely uncomfortable. You hear this bang bang, my mind says nearly kills you. But the good thing, the redeeming things, you walk out. By the time you put your quotes on, put your shoes on, the picture is already on the monitor, right? It's almost immediate. And why is that? It's just an FFT. That's all there is to it. It doesn't require manual, it doesn't require it. It's just fast algorithms and boom. And there's a lot of pictures. I mean, when you do an MRI, I mean, this spine thing, I thought I had like 20, 30 images, right? They cut the discs this way, they take the lateral pictures like this. Anyway, this is an image of a spine of an MRI. And on part B, what you see is enhanced version of this with the power law with sequels one and gamma equals 0.6. And here is gamma equals 0.4 and this is gamma equals 0.3. And I personally think that 0.4 or 0.3 allows you, you see, this is a spine fraction right here, allows you to see the fraction much, much, much better. So here's an example of power law transformation. Moving on, so this image was very dark, so we used small values of gamma. Remember, gamma is less than one, has this kind of characteristic, so it makes the image a little bit brighter. The opposite is happening here is the original image picture you took. And here now, you apply the power law transformation with gamma equals 3 or 4 or 5. And you get this one, this one, this one, as you can see, you can see a lot more detail here, here and here. But by the time you get to this part, these regions have kind of become a little bit too dark. So this is kind of the end of talking about the power law transformation. Are there any questions? Okay, so the next transformation I'm going to talk about is a piecewise linear transformation. And in particular, I'm going to talk about what's called contrast stretching. So piecewise linear transformation basically means is that this is R, again, this is S, you're operating on a pixel-by-pixel basis. And by the way, how do you, in real life, how do you implement this gray-level transformation that transforms one pixel to the other? You just do table lookup, so implementation is extremely straightforward. Anyway, so piecewise linear transformation, you, what you're doing is you have piecewise linear curves like this that makes you, that you design by hand. And I'm sure MATLAB image processing package has a cursor that allows you to design these curves by hand. So this is R1 and S1 is 1, and you define R2 and S2 here, and boom, you've designed yourself a custom-made transformation. And what does it do? Well, it has, this thing has a certain slope for this range of R and then another slope for another range of R and you can make this. Hey Amazon Prime members, why pay more for groceries when you can save big on thousands of items at Amazon Fresh. Shop Prime exclusive deals and save up to 50% on weekly grocery favorites. Plus save 10% on Amazon brands, like our new brand Amazon Saver, 365 by Whole Foods Market, Aplenty, and more. Come back for new deals rotating every week. Don't miss out on savings. Shop Prime exclusive deals at Amazon Fresh. Select varieties. We wear our work, day by day, stitch by stitch. At Dickies, we believe work is what we're made of. So, whether you're gearing up for a new project or looking to add some tried and true work wear to your collection, remember that Dickies has been standing the test of time for a reason. Their work wear isn't just about looking good. It's about performing under pressure and lasting through the toughest jobs. Head over to Dickies.com and use the promo code WorkWear20 at checkout to save 20% on your purchase. It's the perfect time to experience the quality and reliability that has made Dickies a trusted name for over a century. It's more complicated. You can have R2, S2, and then R3, S3 as many as you want. But now the problem is how to design these values. Here's an example of this transformation in action. So, what you see here is the SEM of a pollen, electron microscope of a pollen, and scanning electron microscope. And here is after you apply, excuse me, can you get that the computer? She's talking to herself. Hello. Yeah, I think in this lecture, you really have to pay constant attention because we're going back and forth a lot. Thank you. So, what this is is a transformation. This is a SEM scanning electron microscope of a of a pollen. This is after you apply a transformation of this type to it. And I tell you what that is. It's R1 is equal to R min, which is the smallest intensity value here. And let's see, R2 is R max, which is the largest intensity value here. And S1 is 0, and S2 is L minus 1. And if you do that, you get something like this, which is, we can see, we can see the distinct, they look like beams, but they're not, they're pollens. You can see the distinct pollens, and you can see the dots on them. And this is what you get if you just threshold it. That means that, let's see, R1 is equal to R2, and S1 is equal to 0, and S2 is equal to L minus 1. So, it, oh, I didn't mean to do that. So, it goes from here to a straight line, and then up, and then that way, this is the threshold in that. So, the thresholding is a special case of this piecewise of having a contrast stretching type thing. Another, coming back to the, to the picture, to the, to the paper here, another type of thing that you can do is called gray level slicing. And the characteristic for that is shown, again, coming back to, to the computer, it's shown here. So, essentially what you're doing is you pick a slice in the input, and for that, for that, those range of intensity values at the input, you choose the output intensity to be some, some arbitrary value. Or you could say, you could keep the intensity values up to point A, up to intensity input values point A, and from B onwards, but in the middle, you want to make this thing be brighter, for example. And here's an example of an actual image that does this. And this could be useful in situations where, you know, for example, you have a satellite image, and you know that the water pixel values are in this range, and you want to kind of enhance or boost the water value so it's more apparent, you can see it better, etc. Next is mid-plane slicing, and what mid-plane slicing does is, is exactly what, what you think it does. You have an 8-bit image, and this is the mid-plane number 7 has the most significant bit, the next one, next one, next one, all the way to the least significant one. And mid-plane slicing means that you essentially threshold your image initially with this, and then with this, and then with this, and then with this, and then with this, and with it. And if you, if you initially mid-plane slice it with mid-plane number 7, you would think that it's almost like thresholding it, you're thresholding with different values, but when you threshold it with such a high value, so let's say this is a, this is a fractal image, you threshold it with a very high value, you get something like, like this, this is with 7, bit-plane 7, this is bit-plane 6, 5, 4, 3, 2, 1, and 0. I wish he hadn't done it with the fractal image, I wish he had done it with the natural image. If it was a natural image, and you bit-plane it with the bit-plane number 7, what do you get? You get kind of the black and white, you get what we saw last, last lecture where you kind of get, it's the trash, actually, you know what, I'm going to show it and, and burn your roads, powerpoint, because he has an example of that. Yeah, and for obvious reasons, he, he processed his own, his own image, right? So if you cut it with bit-plane 7, you get something like this, okay? The, the, the, the overall features are shown, and then when you, when you process it with bit-plane number 0, the least significant bit, what do you expect to get? Just noise, right? The least significant bit is can, can be almost anything, it doesn't really correspond to any feature in the image. So, so that's, that's kind of what you would expect this thing to be. So in, in this, in this example, it's coming back here, so this is the fractal image, and this is between 7, 6, 5, 4, 3, 2, 1, 0, and what else do you notice in between these bit-planes? There's a lot of correlation between the patterns, right? Like this is very much correlated with that, this is correlated with this, this, you see this pattern going across, patterns going across, etc. So in general, bit-plane coding of images is not a very efficient way because you, there's a huge amount of correlation between the bit-planes. Okay, any questions? Next, what I want to talk about is histogram processing, and in particular, I'm going to talk about two things. One is called histogram equalization, the other one is called histogram matching. And before I do that, I would like to first explain what a, what a histogram is. So let's switch back to the paper we can. Thank you. So, histogram processing. So what is a histogram of an image? Anybody want to guess? Well, you have an image between that takes on the intensity value between 0 and l minus 1. It's essentially coming over the, and we call the intensity levels n sub k, that range between 0 and l minus 1. So histogram is telling you how many pixels have intensity n 0, how many have n 1, how many have n 2? It's essentially the probability distribution function, the probability of n sub k. It's the probability distribution function of the intensity values of an image. That's, that's called a histogram. Many times we normalize it. So because if these are probabilities, you want the sum of the probabilities to add up to 1. So that's, that's kind of what you do. And I want to motivate this histogram equalization business by the following. We go back to the bean, it's not really bean, it's the pollen, but I keep calling it a bean. It kind of looks like coffee beans to me, but I'm sure pollens are not coffee beans. Pollens are terrible because infinite amount of grease among people. Okay, so here's the, here's the pollen example with the SEMs. That's the original image we had. And, and this is what happens. Sorry, the original image was, was here. Was this one. So you start with that and you apply different power law transformations to get these various images. So this is, this, this is one power law transformation. This is another one, this is another one, and this is another one. And this is the, this, the last one is the one we came up with here that took, took us from this, to this. All right. And next to them, next to each one of these, I'm showing you the, the histograms. So this is this zero, this is 255, 0255, 0255, etc. And as you can remember, expect this is a dark image and the histogram is shifted to the left. This is a light image, bright image. So this histogram is shifted to the right. This is a low contrast image and the, the histogram looks kind of like this. And the image that visually looks the best has kind of what, what kind of histogram does it have? Almost uniform and has a histogram that's uniform. So I hope that this example kind of visually convinces you that having a uniform distribution histogram results in good looking images. Sometimes it doesn't. I mean, I'm not saying it's a uniform universal law, but that, that's a good thing to have. So the basic idea behind histogram equalization is that you start with an image that has this messed up spectrum. And you want, I'm not spectrum. I mean messed up histogram. Sorry. It has a histogram that, that's, that's clumped all in one region of, of, of the intensity space. And the purpose of histogram equalization is to come up with an image from that, that has a, has, if you were to plot its spare histogram, it would look more or less flat. And the question is, how do we go about doing that? And I'm going to do this in, in two steps. First I'm going to do with intuitively with an example. And then I'm going to do it a little bit more mathematically, so you get the idea of what's going on. So intuitively with a, with an example, I have some other pictures here that I'll show you. So here's what we're trying to do, okay? Suppose that if you can zoom in, please, great. Thank you. Suppose that I have a histogram that, that looks something like this. I have an image that consists of pixels between two to, two to five. And this is a really dumb, simple example, just to get the point across. And this is the histogram of the input image. And we want to come up with a transformation. Somehow we've decided that we want the desired histogram of the, after we apply a transformation to this thing, we want to design a transformation that after it's applied to this input image, results in the output intensity that has a histogram like this. And so the question is, how do we go about this? This is piece of n, p of n sub x, the input histogram, and this is p of n sub y, this is the output histogram. And the way to go about this is to move this, this is a probability mass function. This is to move from PMF to CDF, from PDF, probability distribution functions to CDF, cumulative distribution function. What, what do I mean? Well, it's just one is the integral of the other, right? Okay. So this is shown here, right? Do you have an input histogram, piece of, piece of, p of an x, you have a desired output histogram, which is p sub d of an y. So we want to find the transformation. And what we do is from p of n of x, we compute p prime of n of x, which is the summation from k equals zero to seven and, and sub x of p of k. It's essentially the, in the, in the continuous random variable domain, it's just the integral of the probability distribution function. Here, we're taking the sum. So the probability distribution function, if I were to write here, PDFs, generally speaking, look like this, the area under PDFs are as always if I integrate the area under probably distribution function, I always get one. So if I were to integrate this function and come up with CDF, it starts at zero. Hey Amazon Prime members, why pay more for groceries when you can save big on thousands of items at Amazon Fresh? Shop prime exclusive deals and save up to 50% on weekly grocery favorites. Plus save 10% on Amazon brands, like our new brand Amazon Saver, 365 by Whole Foods Market, a plenty and more. Come back for new deals rotating every week. Don't miss out on savings. Shop prime exclusive deals at Amazon Fresh. Select varieties. We wear our work day by day, stitch by stitch. At Dickies, we believe work is what we're made of. So whether you're gearing up for a new project or looking to add some tried and true work wear to your collection, remember that Dickies has been standing the test of time for a reason. The work where isn't just about looking good. It's about performing under pressure and lasting through the toughest jobs. Head over to Dickies.com and use the promo code Workware20 at checkout to save 20% on your purchase. It's the perfect time to experience the quality and reliability that has made Dickies a trusted name for over a century and it goes all the way to one. It's a monotonically increasing function. So this is P of N of X and this is P prime of N of X. The CDF of this, the integral P of N of X, the N of X. So coming back here, so I start with P and I get P prime which is the CDF of P and I do this, I play the same game at the output in a sense that I have been given a desired probability distribution function for my output image, P sub D of N sub Y. N sub Y is the intensity values of the output, D stands for desired and from that probability distribution function, I build a communicative distribution function CDF from PDF and that's another integral. So now I have P prime of N sub X and P prime D of N sub Y. Now I'm going to choose a transformation that maps this guy into this guy. And how do I do that mapping? Well, again, I'll show it to you with an example and this is shown here. So suppose I have an input histogram like this and suppose somehow I've decided that I want the output histogram to look like this. And I first build the communicative input histogram by integrating this and integrating this function gives me this function, monitoring to increasing from 0 to 64. Sorry, from 0, the input intensity is from 0 to 16, always 0 to 16, 0 to 16 in case you can't read it. And the P prime of N of X goes from 0 all the way to 1 and I don't know why this axis says 64, just ignore that. And this is my desired output histogram, it looks like this and I integrate that and I get this function. Oh, I know what it's what it's 64. This is for an 8 by 8 image, so the 64 pixels. And so here, for example, we have four pixels that have intensity value 0, six pixels that have intensity value 1. So when I come here, I have four pixels that have intensity value 4 or smaller than 4. And by the time I get to 1, I have six pixels that I didn't have intensity value 1 and 4 that have intensity value 0. So I have 10 pixels that have intensity value of 1 or lower. And so at a particular pixel point here, like 12, this the number here tells me like it's 60, can't read this variable 63. That means that 63 out of my 64 pixels have intensity values of 12 or lower. And by the time I get up here, it's all 64. That means all 64 of my pixels values have intensity of 15 or lower. Big deal, we already knew that, right? So it goes in the probability domain, it goes from 0 to 1. In the histogram domain, it goes from 0 to 64. Clear to everyone? Okay, now I have to find the transformation between these two things. This is the desired, this is the actual. And the way I kind of do that is the following. I say, okay, I want to know, for example, input intensity 6 in my input image, what output intensity pixels should it get mapped to? So what I do is I start from 6 in this CDF, and I go all the way up, and I look at the height of this thing. The height of this thing is 48. That means in the input Instagram, 48 pixels have intensity value 6 or lower. So I look up this number 48, and I come back in this picture, and I say, what output intensity should I move around until I hit 48? And so I go to this axis here and look up 48, and wow, 48 is here, I go all the way down, and the output intensity is 10. So input intensity 6 gets mapped to 10. How about intensity 12? Well, 12 has a height of 63, and if I go to 63 here, it's 14. So 12 gets mapped to 14. How about 3? 3 has a height of 29, and I come back here and I find 29, and it's somewhere between 6 and 7, so it gets mapped to 6 and a half. So during that process, I can find the input-output relationship between my input intensities and output intensities. Is that clear? And by the virtue of the way I did this, after I applied this transformation that I just obtained the way I just explained, if I now apply this to the input histogram, I'm going to get something that is supposed to look a little bit to look like the desired, and it kind of does. So if I apply, so I build this transformation using the steps I just described, I apply this transformation to the input histogram, and what comes out is output histogram like this, which almost looks like this. And you might start complaining, can you zoom out just a little bit? Thank you. And you might start complaining and saying, oh, a little bit more out. I want both of these to be shown. Okay, great, thank you. So you might start complaining, well, this thing doesn't really look like that. Well, first of all, this looks a lot more like this, looks a lot more like this than the input histogram, so that's good. And the reason there's some points in the middle is because there's discretization going on. You can't hit things precisely. Okay. So in this case, even one step further beyond what I told you, I told you how to do, how to change the histogram of an input to an arbitrary desired output histogram. And let me just show you an example of this thing, first from Jay Len's book, and then from some other examples. So if I look at picture, oh, you know what? I could have shown it on these, but it doesn't matter. So here's, can you zoom in, please? Here's an input image. I assume you don't see much, right? And here's the histogram corresponding to that input image, all clumped together near low intensity values. And I applied the processor, just described, I get this transformation, and you can see the transformation, the input range, can you focus this a little bit? Because the book has certain thickness, so the lens has to, has to be adjusted a little bit. Okay, good, good. Anyway, the input, the input intensity ranges between 0 and 100, but this transformation you can see is going to map that 0 to 100 to 0 to 250. And after I apply this transformation to this image, I get this image. It's a lot nicer. Okay, and here's another example of a wedding picture. Less dramatic, this becomes that. I don't think this character, this shows very well in the monitor. So what I like to do now is actually switch back to get a different PowerPoint, because I think that has the best set of pictures. And show you other examples of histogram modification. Here we go. Okay, so here's a histogram of the picture called camera man. We already talked about that a little bit. And as you can see, it has a, it has a, this sort of a shape that's a little bit bumpy. And here's an histogram of the pout image. Is that put or pout? Po, and what does it mean something? Oh, good. I learned a new word today. Okay, so pout image of an upset kid. And as you can see, the range of the intensity values are shown here. And after, I'll go over this mathematics in just a second. Hold on, hold on. It's actually quite easy. You already explained it to you. So the intuitive explanation covers all of that. But we'll go over the mathematics in just a second. So here's, here's the output hist image after you've applied histogram equalization to this. So it looks a lot nicer. And I hope that he has a, he shows the histogram of the output as well. I sincerely hope so. Yeah, he does. So, so the, the, the, un-equalized histogram looks like this. And the equalized one is a lot, it stands a lot bigger range. And it covers a lot bigger values of intensity. And, and this is the best image to take a look at. Does this come across on your monitor? Just a minute. Actually, what I'm seeing on the screen is so much better than what you have. I think, I wish we had those, those are projectors. The, what do you call those? The ones that we have for PowerPoint presentation, pun. It has, it's not LCD, but it has other name as well. Okay. Yeah. Oh, the, the, that's exactly right. That's the Texas instrument part, right? Very good, very good. It's, it's an array of micro mirrors, by the way, that, that, that's the stuff that, that, that send the works on. So, okay. So, so we saw that. Let's, let's look at another histogram and equalization example. This is before, and this is after. You could argue which actually looks better. I would say you see a little bit more detail here, but this region has become too white, in my opinion. So, and this is before and after for the cameraman texture. Okay. So, before you have a bump here, it doesn't span the entire range. Now, we, we have a much, much bigger range and just, just so that not that you've seen the histograms, let's look at the image one more time. Yeah. This is a lot less dramatic than the, than the put image, pouch image. Sorry. Okay. And let me see, he had few more good examples. Okay, here we go. This is a original image of the moon. This is after histogram equalization. There's a lot more noise in there, in, in here, but you can hopefully see a little bit more detail there. I don't know. I, that's not a very convincing example. I, I don't, I don't think so. This was before. This is the histogram before. Now, this is the histogram after. Actually, we'll show you a picture of a moon in, in next time's lecture that histogram equalization is actually a bad idea. And it's much better not to equalize the histogram, but change the histogram according to a desired one that, that gives you nice characteristics. I don't know if Bern was going to do, no, he's not going to do that. Okay. Enough of, uh, Stanford pictures. Um, do I have time? No, I think if I start going on the mathematical derivation, we want all the time. And then I have to restart it again next time. But let me, well, while I'm at it, let me also just, just show you one more picture off of this book. And then next time's lecture, I just quickly do the mathematical derivation. Um, here. So here's the bean picture. It's, it's really the pollen. I keep calling it a bit. It's, uh, on the left, you see various images. And on the right, what you see is, um, the histogram equalized, uh, images resulting from these. So if it is too dark, too, too light, everything that goes wrong, if you do the right histogram equalization, not only do you get more of a flat histogram, uh, the resulting images all look good. And note that even though the histogram, these are the histograms of the resulting images, even though the histograms of these resulting images are not identical to each other. And they can't be. They all look visually good. And even if you started with a good image, it kept it as a good image. It didn't mess it up, which is an important property of your image processing algorithms. And these are the four input output characteristics, uh, input output transformations for these four examples here. So what I'm going to talk about next time is, is just, uh, you know, a little mathematical derivation of that, and then talk about, um, the rest of our discussion on image enhancement. Don't forget to pick up your, um, greatest homework and the solutions and all that. Hey Amazon Prime members. Why pay more for groceries when you can save big on thousands of items at Amazon Fresh? Shop Prime exclusive deals and save up to 50% on weekly grocery favorites. Plus save 10% on Amazon brands, like our new brand Amazon Saver. 365 by Whole Foods Market, a plenty and more. Come back for new deals rotating every week. Don't miss out on savings. Shop Prime exclusive deals at Amazon Fresh. Select varieties. When you need meal time inspiration, it's worth shopping king supers for thousands of appetizing ingredients that inspire countless mouthwatering meals. And no matter what tasty choice you make, you'll enjoy our everyday low prices plus extra ways to save, like digital coupons worth over $600 each week and up to $1 off per gallon at the pump with points. So you can get big flavors and big savings king supers fresh for everyone. Fewer restrictions apply.